Artificial intelligence could help create transparency and consistency in the legal system – our model shows how.
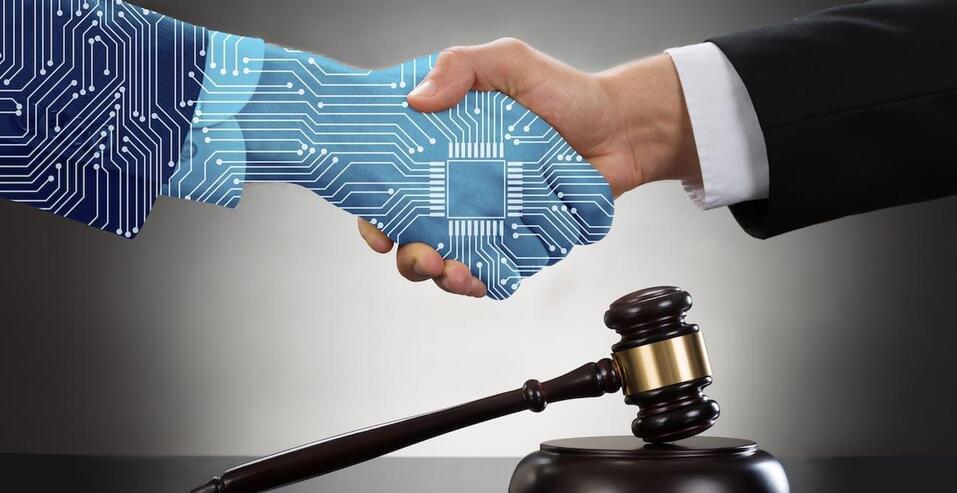
In our cells, the language of DNA is written, making each of us unique. A tandem repeat occurs in DNA when a pattern of one or more nucleotides—the basic structural unit of DNA coded in the base of chemicals cytosine ©, adenine (A), guanine (G) and thymine (T)—is repeated multiple times in tandem. An example might be: CAG CAG CAG, in which the pattern CAG is repeated three times.
Now, using state-of-the-art whole-genome sequencing and machine learning techniques, the UNC School of Medicine lab of Jin Szatkiewicz, Ph.D., associate professor of genetics, and colleagues conducted one of the first and the largest investigations of tandem repeats in schizophrenia, elucidating their contribution to the development of this devastating disease.
Published in the journal Molecular Psychiatry, the research shows that individuals with schizophrenia had a significantly higher rate of rare tandem repeats in their genomes—7% more than individuals without schizophrenia. And they observed that the tandem repeats were not randomly located throughout the genome; they were primarily found in genes crucial to brain function and known to be important in schizophrenia, according to previous studies.
The goal of achieving what is called artificial general intelligence — or the capacity of an engineered system to display human-like general intelligence — is still some time off into the future. Nevertheless, experts in the field of AI have no doubt accomplished some major milestones along the way, including developing AI capable of deep neural reasoning, tactile reasoning, and even AI with rudimentary social skills.
Now, in yet another step toward AI with more human-like intelligence, researchers from IBM, the Massachusetts Institute of Technology and Harvard University have developed a series of tests that would evaluate an AI’s ability to use a machine version of “common sense” — or a basic ability to perceive, understand, and judge in a manner that is shared by nearly all humans.
@Meet Kevin is a 30-year-old dad and financial analyst. He’s amassed a following of nearly 2 million subscribers on YouTube with his large library of financial content. He recently ran for California governor and owns a lot of Tesla stock.
Meet Kevin’s YouTube: https://www.youtube.com/@MeetKevin.
Neura Pod is a series covering topics related to Neuralink, Inc. Topics such as brain-machine interfaces, brain injuries, and artificial intelligence will be explored. Host Ryan Tanaka synthesizes informationopinions, and conducts interviews to easily learn about Neuralink and its future.
Twitter: https://www.twitter.com/ryantanaka3/
Support: https://www.patreon.com/neurapod/
Please consider supporting by joining the channel above, or sharing my other company website with retirees: https://www.reterns.com/. Opinions are my own. Neura Pod receives no compensation from Neuralink and has no affiliation to the company.
You can’t discuss fulfillment robots without mentioning Amazon. Over the past decade, the retail juggernaut has become the 800-pound gorilla in the category, courtesy of several key acquisitions and seemingly endless resources. And while warehouse robotics and automation have been accelerated amid the pandemic and resulting employment crunch, Amazon Robotics has been driving these categories for years now.
This week at its annual Re: Mars conference in Las Vegas, the company celebrated a decade of its robotics division, which was effectively born with its acquisition of Kiva Systems. Over the course of its life, Amazon Robotics has deployed more than 520,000 robotic drive units, across its fulfillment and sort centers. From the outside, it’s been a tremendous success in the company’s push toward same-and next-day package delivery, and its driven the competition to look for their own third-party robotics solutions, bolstering startups like Locus, Fetch and Berkshire Grey.
The team replicated different patterns of materials and found arrangements that would let water through more easily.
Artificial intelligence (AI) has been found to be useful in the creation of water filter materials and can quicken the process involved in making them, according to a study published today (Nov .30) in the journal ACS Central Science.
Creating a novel water purification system
From daily household faucet attachments to room-sized industrial systems, filter systems are used in a variety of items. However, it is difficult for current filtration membranes to filter water if the water is extremely dirty or has small, neutral molecules, such as boric acid, an insecticide used on crop plants.
The organization says the machines would only be used in extreme situations where lives are at stake.
Supervisors in San Francisco voted Tuesday to allow city police to use potentially lethal remote-controlled robots in emergency situations, according to a report by Mission Local.
A dystopian future?
Onfokus/iStock.
The vote was eight for three against, with opponents saying the move would lead to the further dangerous and unwanted militarization of a police force already too aggressive with minorities.
Artificial intelligence would be used to detect changes in the vocals of each patient after a laryngectomy.
Researchers from Kaunas University of Technology Faculty of Informatics (KTU IF) and Lithuanian University of Health Sciences (LSMU) in Lithuania have created a new substitute voice evaluation index that can detect pathologies in patients’ voices more quickly and efficiently. Voice pathologies include a variety of disorders such as growths on the vocal cords, spasms, swelling or paralysis in the vocal cords.
AI could be used to determine changes in voice after laryngectomy.
Laryngectomy is a surgery that requires the removal of the larynx.
Simarik/iStock.
“For some, the voice changes only slightly, while for others, it can be a life-changing situation. Imagine calling someone on the phone, emergency services, police, etc. – and the one you’re calling does not understand anything. Or even not hear you – as the phone’s noise removal system will cut it out,” said Dr. Rytis Maskeliunas, professor with the Department of Multimedia Engineering, Faculty of Informatics and chief researcher at Kaunas University of Technology Faculty of Informatics.
It is the first ever commercial partnership between Google Health and the medical company iCAD.
Google recently announced that it licensed its AI research prototype to a medical company called iCAD. The AI research model can be used for breast cancer screening. iCAD is a company that creates innovative medical equipment for cancer detection.
ICAD made the announcement about the collaboration yesterday, Nov. 28, on its website.
It is the first time ever that Google Health has licensed this specific artificial intelligence technology in a commercial partnership for breast cancer screening and personalized risk assessment of the disease, to iCAD.
ChooChin/iStock iCAD made the announcement about the collaboration yesterday, Nov. 28, on its website.