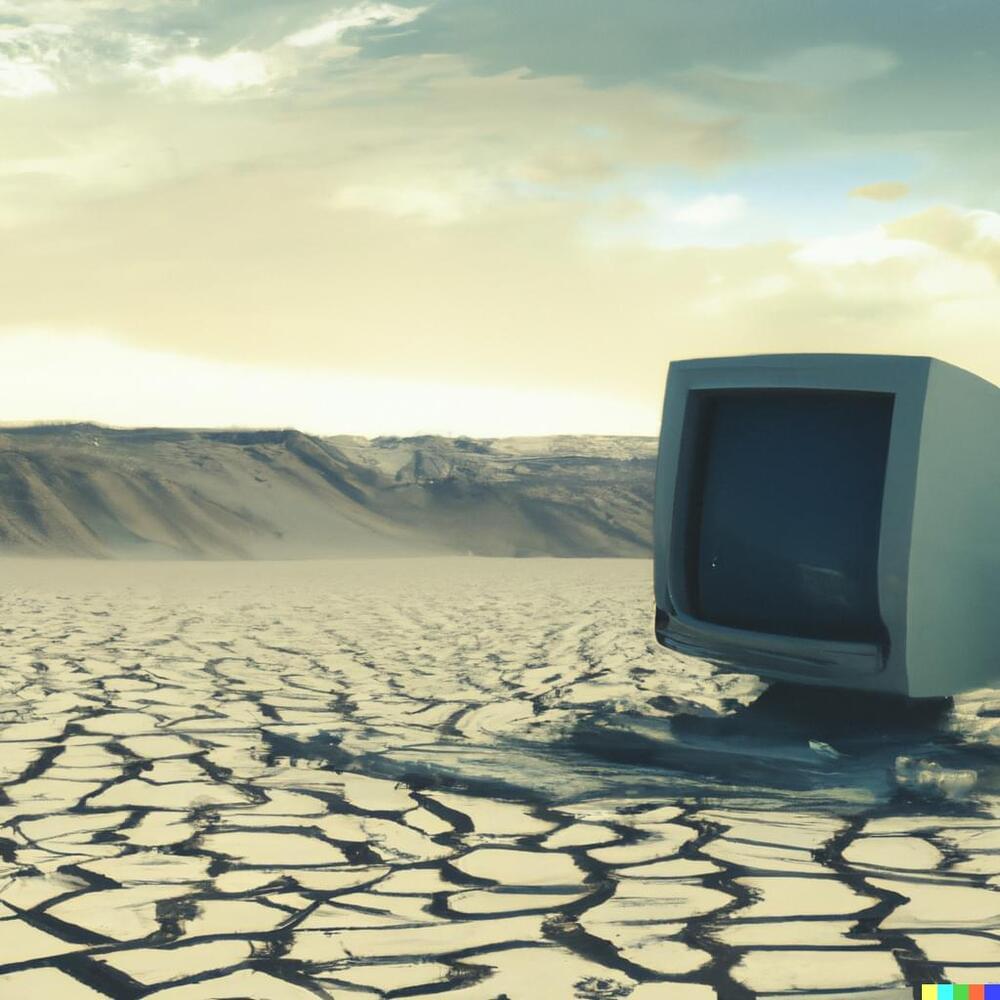
The end of classical Computer Science is coming, and most of us are dinosaurs waiting for the meteor to hit.
I came of age in the 1980s, programming personal computers like the Commodore VIC-20 and Apple ][e at home. Going on to study Computer Science in college and ultimately getting a PhD at Berkeley, the bulk of my professional training was rooted in what I will call “classical” CS: programming, algorithms, data structures, systems, programming languages. In Classical Computer Science, the ultimate goal is to reduce an idea to a program written by a human — source code in a language like Java or C++ or Python. Every idea in Classical CS — no matter how complex or sophisticated — from a database join algorithm to the mind-bogglingly obtuse Paxos consensus protocol — can be expressed as a human-readable, human-comprehendible program.
When I was in college in the early ’90s, we were still in the depth of the AI Winter, and AI as a field was likewise dominated by classical algorithms. My first research job at Cornell was working with Dan Huttenlocher, a leader in the field of computer vision (and now Dean of the MIT School of Computing). In Dan’s PhD-level computer vision course in 1995 or so, we never once discussed anything resembling deep learning or neural networks—it was all classical algorithms like Canny edge detection, optical flow, and Hausdorff distances. Deep learning was in its infancy, not yet considered mainstream AI, let alone mainstream CS.