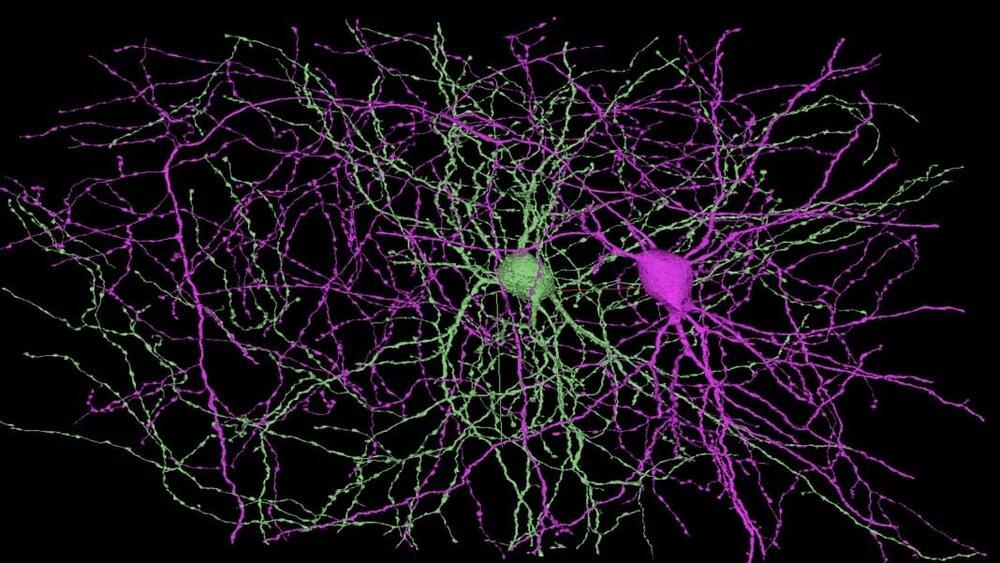
It’s easy to see why: as shockingly powerful mini-processors, neurons and their connections—together dubbed the connectome—hold the secret to highly efficient and flexible computation. Nestled inside the brain’s wiring diagrams are the keys to consciousness, memories, and emotion. To connectomics, mapping the brain isn’t just an academic exercise to better understand ourselves—it could lead to more efficient AI that thinks like us.
But often ignored are the brain’s supporting characters: astrocytes—brain cells shaped like stars—and microglia, specialized immune cells. Previously considered “wallflowers,” these cells nurture neurons and fine-tune their connections, ultimately shaping the connectome. Without this long-forgotten half, the brain wouldn’t be the computing wizard we strive to imitate with machines.
In a stunning new brain map published in Cell, these cells are finally having their time in the spotlight. Led by Dr. H. Sebastian Seung at Princeton University, the original prophet of the connectome, the map captures a tiny chunk of the mouse’s visual cortex, less than 1,000 times smaller than a pea. Yet jam-packed inside the map aren’t just neurons; in a technical tour de force, the team mapped all brain cells, their connections, blood vessels, and even the compartments inside cells that house DNA and produce energy.