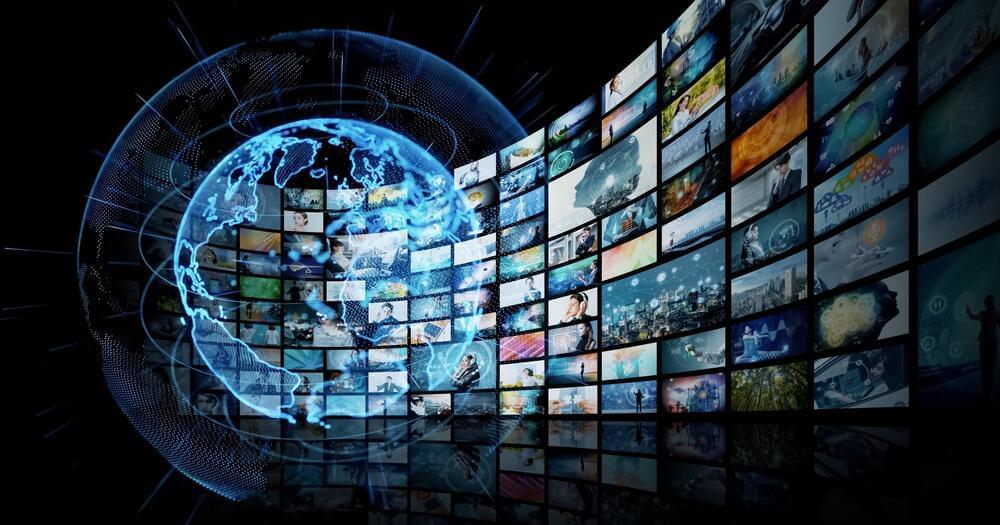
OpenAI’s ChatGPT has taken the world like wildfire and continues to make headlines. However, the Generative Artificial Intelligence (GAI) has been around for a very long time. The technology was first pioneered in academia with Ian Goodfellow and Yoshua Bengio publishing their first seminal work on Generative Adversarial Networks in 2014 and then Google picked up the torch and published seminal papers and patents in both GANs and generative pre-trained transformers (GPT). In fact, my first paper on generative chemistry, was published in 2016, first granted patent in 2018, and the first AI-generated drug went through the first phase of clinical trials.
Forbes is one of the most reputable content providers on the planet and probably the most reputable when it comes to anything dealing with money. If Forbes does not classify you as a billionaire, you are not a billionaire. It has decades of high-quality expert-generated longitudinal text, and multimedia content in multiple languages. In addition to elite human reporters and editors, it also has a small army of content creators specializing in specific areas contributing to Forbes.com. For example, it is my 5th year as a contributor and I contribute regularly to keep the pencil sharp. This massive human intelligence may be partly repurposed to help develop internal generative resources within the Forbes empire, help curate the datasets and help train or benchmark third-party generative resources. I would gladly volunteer a small amount of time to such a task.
Nature and several other journals in the Nature Publishing Group portfolio are considered to be the Olympus in academic publishing. To publish in one of the elite Nature journals academics spend months and sometimes years going through the rounds of editorial and then peer-review. The quality of the data is questioned, all experimental data is disclosed, and the thousands or millions of dollars that went into the experiments are presented in the form of a paper and supplementary materials.
Having vast amounts of highest-quality data that is not available to the public gives Nature and other publishers the ability to either develop their own versions of ChatGPT, sell or license the data, and restructure the editorial and review processes to create more value for the future generative systems.