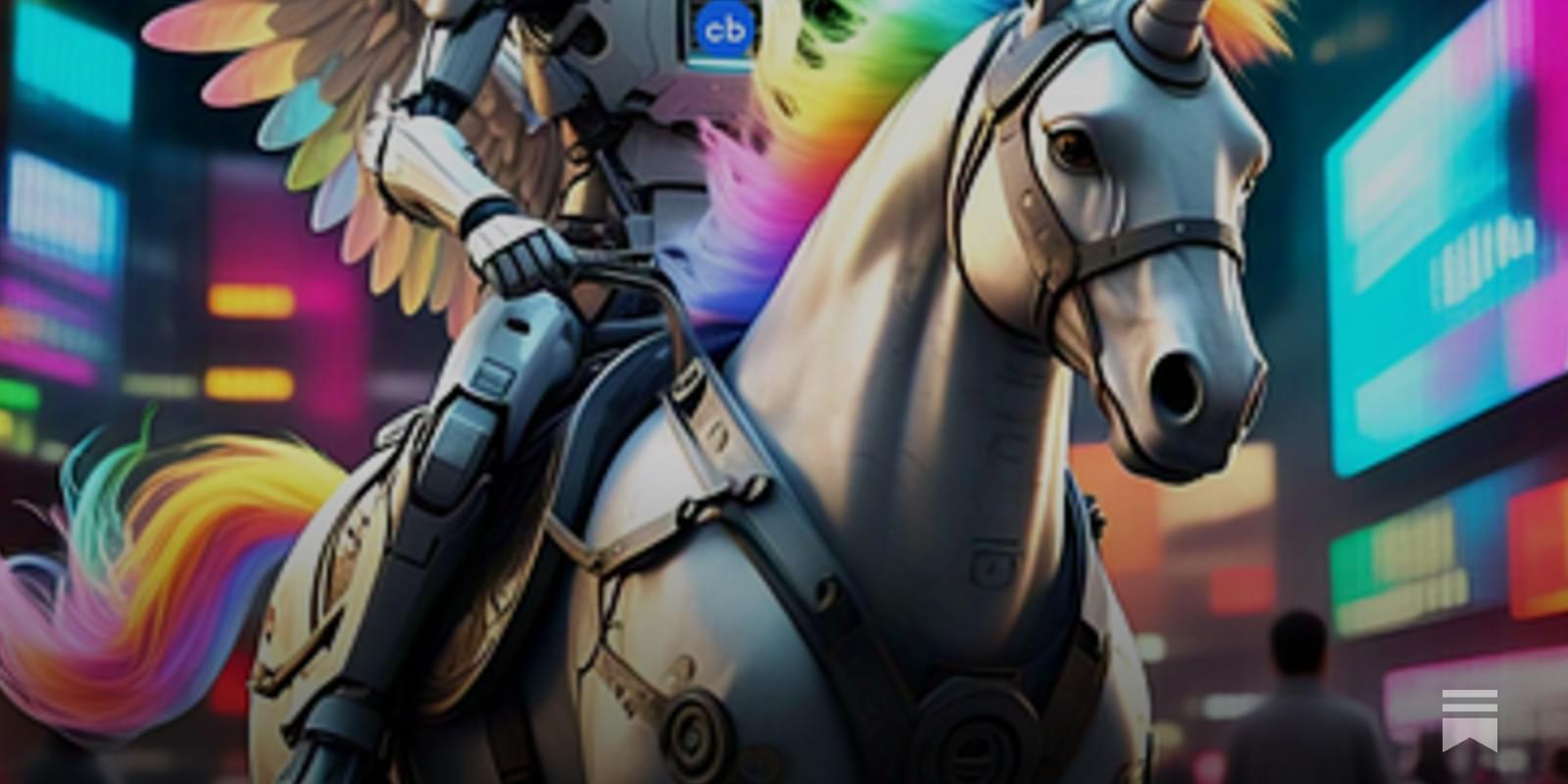
In today’s AI news, Crunchbase, long known as a go-to platform for company data, has relaunched as an AI-powered solution, revolutionizing how investors, founders, and innovators gain insights into private companies. Moving beyond historical data, the new Crunchbase introduces live, predictive intelligence, providing a real-time, forward-looking view of the market. Users can now anticipate funding rounds, acquisitions, and even IPO’s.
In other advancements, EcoDataCenter, a Swedish company that builds eco-friendly data centers used by major compute providers to handle their AI traffic, has raised nearly half a billion dollars — $478 million (€450 million) to be exact — in anticipation of more demand. The equity funding, which is coming from a group of unnamed institutional investors, will be used to continue developing new technologies for more “green” data centers.
Meanwhile, we still didn’t get a straight-up definition of exactly what an AI agent is during Bret Taylor’s Mobile World Congress fireside chat in Barcelona on Tuesday. The Sierra founder and OpenAI board chair preferred to sidestep CNN moderator Anna Stewart’s question asking how “agentic AI” is “any different to a GenAI chatbot” by suggesting everyone hates the former but is delighted by the “empathetic” responses AI agents can serve up.
And, former Google CEO Eric Schmidt, Scale AI CEO Alexandr Wang, and Center for AI Safety Director Dan Hendrycks said that the U.S. should not pursue a Manhattan Project-style push to develop AI systems with “superhuman” intelligence, also known as AGI. The paper, titled “Superintelligence Strategy,” asserts that an aggressive bid by the U.S. to exclusively control superintelligent AI systems could prompt fierce retaliation from China.
In videos, as we join this episode of the Lightcone podcast we find CEO, Garry Tan and the team talking about how Andrej Karpathy recently coined the term “vibe coding” to describe how LLMs are getting so good that devs can simply “give in to the vibes, embrace exponentials, and forget that the code even exists.” They dive into this new way of programming and what it means for builders in the age of AI.