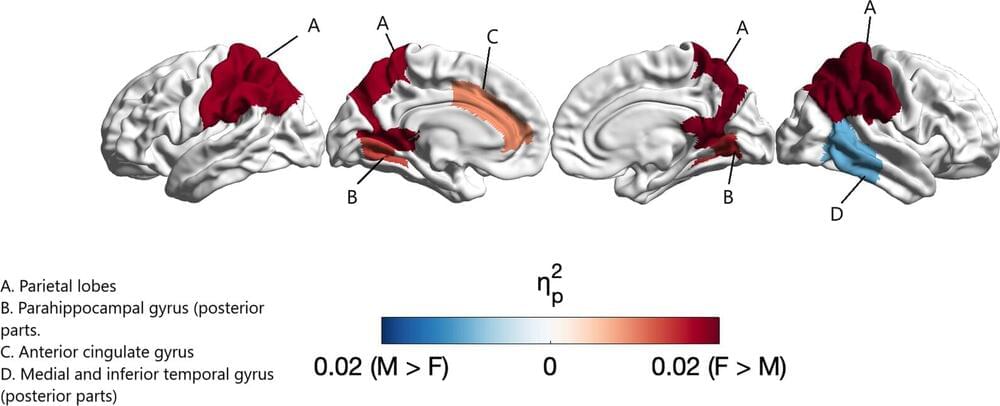
Gray matter is made up of neuron cell bodies and dendrites and is responsible for processing and interpreting information, such as sensation, perception, learning, speech, and cognition. White matter is made up of axons, which are long nerve fibers that connect neurons together from different parts of the brain.
In the study, male brains tended to be greater in volume than female brains. When adjusted for total brain volume, female infants on average had significantly more gray matter, while male infants on average had significantly more white matter in their brains.
Yumnah Khan, a Ph.D. student at the Autism Research Center at the University of Cambridge, who led the study, said, Our study settles an age-old question of whether male and female brains differ at birth. We know there are differences in the brains of older children and adults, but our findings show that they are already present in the earliest days of life.