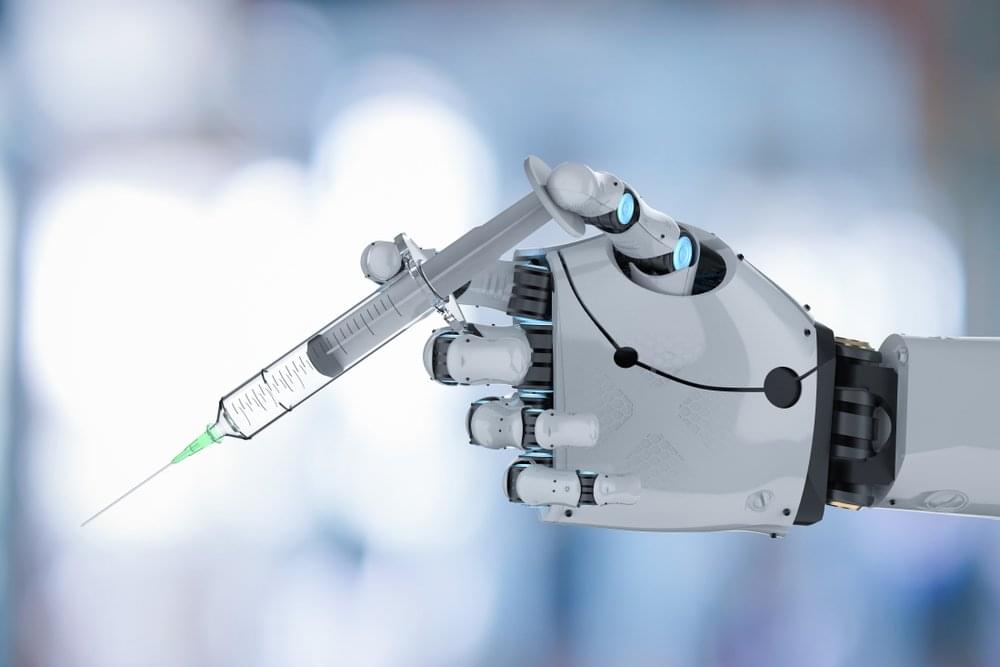
Healthcare start-up Tennr reports a $37 million Series B fundraising round – nine months after raising an $18 million Series A funding round. The young company plans to use machine learning in order to improve patient record keeping, prevent medical error and reduce waiting times for patients. The Series B round was led by Lightspeed Ventures, together with existing investors Andreessen Horowitz and Foundation Capital, and raises the total amount of money raised by the company to $61 million.
Several US healthcare providers have already begun working with the firm, both private doctors’ practices and major clinics and hospitals. These providers receive referrals from primary care providers in different formats to register patients and document their case history. Since providers often compete with each other for patients, there is no standard format used in the industry nationwide, with many companies relying on handwritten documents, messages from private email accounts, and some even using such outdated technology as fax machines. This causes significant delays in the provision of treatment, and increases the likelihood that patients will be misdiagnosed, referred to the wrong clinic or denied access to a specialist whose expertise they require.
Tennr has made it its mission to solve these problems by automating this process: it extracts the relevant information from referrals, no matter what form they’re received in or what technology was used to generate the documents, which not only enables more rapid response times but also creates an unprecedented level of standardization in the medical field, nationally and in the future perhaps globally as well. The company has already processed tens of millions of referrals for patients in the USA, ensuring an appointment with a specialist in a few hours, instead of having to wait several weeks and at times months.