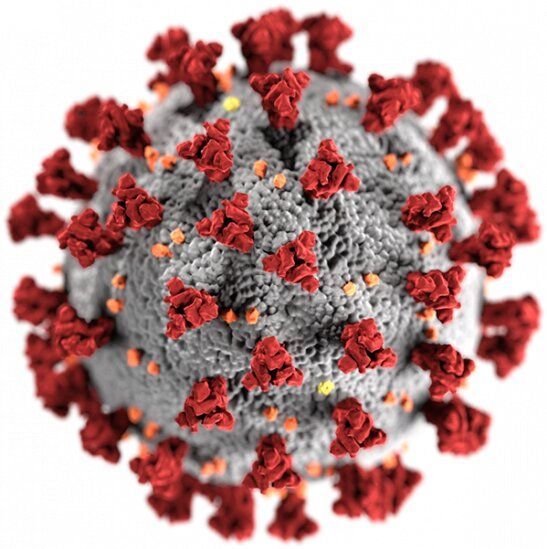
Researchers remain perplexed as to why some patients infected with SARS-CoV-2, the virus responsible for COVID-19, remain asymptomatic while other patients develop severe disease symptoms. This question is once again at the front of mind as the Delta variant spreads across the country. In a new retrospective study, researchers at the Medical University of South Carolina (MUSC) discovered a specific and sensitive biomarker in blood samples that predicts which patients will develop COVID-19 symptoms. Their results, published online on July 9 in Scientific Reports, show that reduced levels of a specific lipid, sphingosine, are significantly associated with developing COVID-19 symptoms. Conversely, elevated levels of sphingosine, as well as a protein involved in its production, acid ceramidase (AC), are associated with asymptomatic infections.
“We developed this project at a time when there wasn’t a successful vaccine,” said Besim Ogretmen, Ph.D., director of the Lipidomics Shared Resource at Hollings Cancer Center and leader of the Hollings Developmental Cancer Therapeutics Research Program. “We wanted to contribute to the field and know which patients who were exposed to this virus would be symptomatic versus asymptomatic.”
Over the past 16 months several waves of SARS-CoV-2 infections in the U.S. have resulted in more than 35 million cases and almost 630000 deaths. Despite the development of multiple safe and effective vaccines, we are currently experiencing another wave of infections.