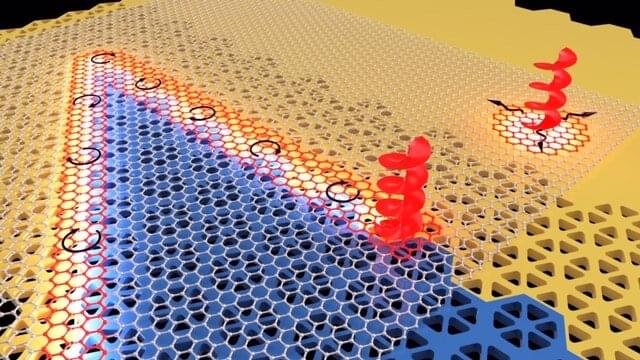
New research by a City College of New York team has uncovered a novel way to combine two different states of matter. For one of the first times, topological photons—light—has been combined with lattice vibrations, also known as phonons, to manipulate their propagation in a robust and controllable way.
The study utilized topological photonics, an emergent direction in photonics which leverages fundamental ideas of the mathematical field of topology about conserved quantities—topological invariants—that remain constant when altering parts of a geometric object under continuous deformations. One of the simplest examples of such invariants is number of holes, which, for instance, makes donut and mug equivalent from the topological point of view. The topological properties endow photons with helicity, when photons spin as they propagate, leading to unique and unexpected characteristics, such as robustness to defects and unidirectional propagation along interfaces between topologically distinct materials. Thanks to interactions with vibrations in crystals, these helical photons can then be used to channel infrared light along with vibrations.
The implications of this work are broad, in particular allowing researchers to advance Raman spectroscopy, which is used to determine vibrational modes of molecules. The research also holds promise for vibrational spectroscopy—also known as infrared spectroscopy —which measures the interaction of infrared radiation with matter through absorption, emission, or reflection. This can then be utilized to study and identify and characterize chemical substances.