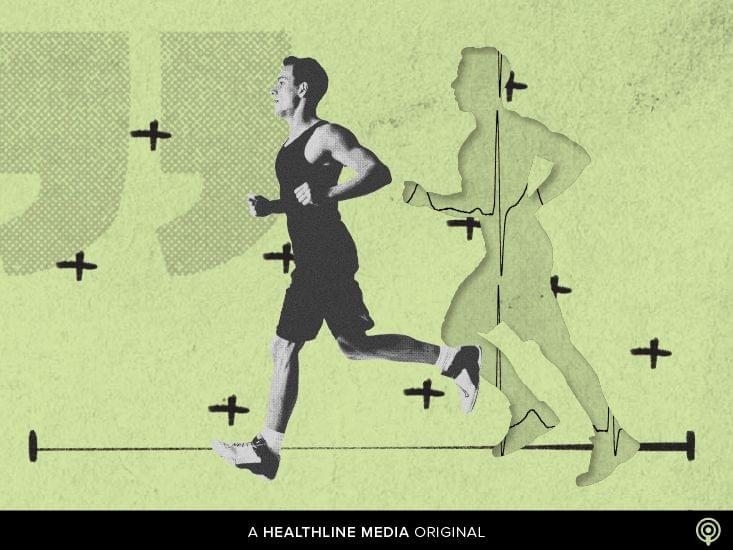
In this episode of In Conversation, we turn the focus to all things extreme exercise and longevity. Based on the findings of a recent study, which found that a select group of elite runners could live around five years longer on average than the general population, Medical News Today editors Maria Cohut and Yasemin Nicola Sakay discuss the probable biological mechanisms behind how more extreme forms of exercise, such as 4-minute mile running, affect longevity with an expert in cardiology.
Joining the conversation is Michael Papadakis, president of the European Association of Preventive Cardiology (EAPC), professor of cardiology at St George’s, University of London, honorary consultant cardiologist at St George’s University Hospitals National Health Service Foundation Trust, and consultant cardiologist at Cleveland Clinic London in the United Kingdom.
Papadakis shares easy-to-follow advice on how to incorporate more physical activity into our daily lives while discussing the potential health risks and benefits of running and similar forms of professional athletic performance.