A humanized antibody from a recently-developed mouse model potently neutralizes SARS-CoV-2 variants by inhibiting membrane fusion.
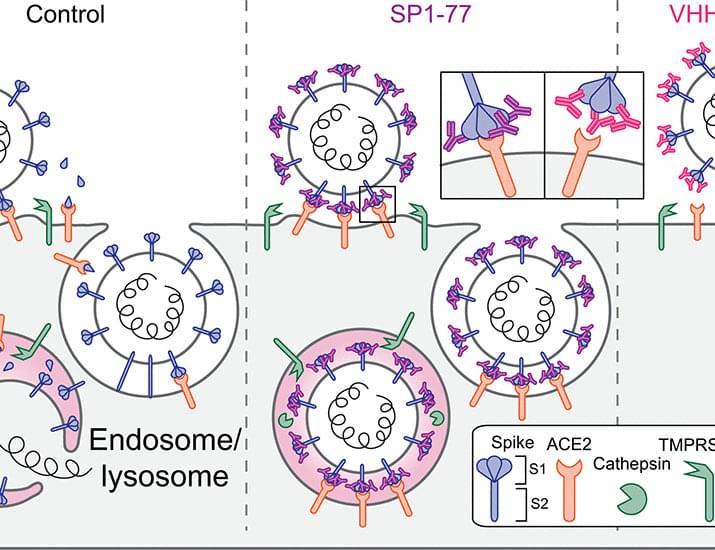
On 30 January 2020 COVID-19 was declared a Public Health Emergency of International Concern (PHEIC) with an official death toll of 171. By 31 December 2020, this figure stood at 1 813 188. Yet preliminary estimates suggest the total number of global deaths attributable to the COVID-19 pandemic in 2020 is at least 3 million, representing 1.2 million more deaths than officially reported.
With the latest COVID-19 deaths reported to WHO now exceeding 3.3 million, based on the excess mortality estimates produced for 2020, we are likely facing a significant undercount of total deaths directly and indirectly attributed to COVID-19.
COVID-19 deaths are a key indicator to track the evolution of the pandemic. However, many countries still lack functioning civil registration and vital statistics systems with the capacity to provide accurate, complete and timely data on births, deaths and causes of death. A recent assessment of health information systems capacity in 133 countries found that the percentage of registered deaths ranged from 98% in the European region to only 10% in the African region.
Even more daring, biology’s “mirror dimension” may be a springboard to engineer synthetic life forms that exist outside of nature, but are literal reflections of ourselves. To rephrase: building a mirror-image version of biology means rewriting the fundamental operating system of life.
Sound a bit too sci-fi? Let me explain. Similar to how our left hand can’t wear a right-hand glove, the building blocks of life—DNA, RNA, and proteins—are etched into specific 3D structures. Flip them around, as if reflected by a mirror, and they can no longer function inside the body. Scientists aren’t yet sure why nature picked just one shape out of two potential mirror images. But they’re ready to test it out.
A new study in Science made strides by reworking parts of the body’s protein-making machine into its mirror image. At the center is a structure called the ribosome, which intakes genetic code and translates it into amino acids—the Lego blocks for all proteins. The ribosome is an iconic cellular architecture, fused from two main molecular components: RNA and proteins.
Summary: Female mice who have not been pregnant or given birth show activation in the anterior cingulate cortex when they acquire maternal behaviors after exposure to pups. The findings reveal through repeated exposure to pups, virgin female mice are capable of learning maternal behaviors that resemble those of mothers following birth.
Source: Medical University of Vienna.
Various conditions such as postpartum depression or postpartum psychosis can lead to an alteration in maternal behavior and disrupt the mother-child bonding process.
THE most impressive designs for near-future Mars bases have finally been revealed.
These elaborate celestial plans are the difference between human life surviving on Mars – and thriving.
When it comes to planning how to live on a planet like Mars, 3D printing has provided scientists with the easiest way of navigating an environment that has similarities, but ultimately boasts a vastly different environment from Earth.
Get a Wonderful Person Tee: https://teespring.com/stores/whatdamath.
More cool designs are on Amazon: https://amzn.to/3wDGy2i.
Alternatively, PayPal donations can be sent here: http://paypal.me/whatdamath.
Hello and welcome! My name is Anton and in this video, we will talk about unusual discoveries coming directly from within our mouths — biofilm complexity.
Links:
https://en.wikipedia.org/wiki/Biofilm.
https://www.pnas.org/doi/full/10.1073/pnas.2209699119
https://en.wikipedia.org/wiki/Quorum_sensing.
Slime: https://www.youtube.com/watch?v=spZwZLkMsYw.
Biofilm communication and bacterial cities: https://youtu.be/4M872c27bSc.
#biology #dentistry #biofilm.
Support this channel on Patreon to help me make this a full time job:
https://www.patreon.com/whatdamath.
Bitcoin/Ethereum to spare? Donate them here to help this channel grow!
bc1qnkl3nk0zt7w0xzrgur9pnkcduj7a3xxllcn7d4
or ETH: 0x60f088B10b03115405d313f964BeA93eF0Bd3DbF
Space Engine is available for free here: http://spaceengine.org.
Enjoy and please subscribe.
Twitter: https://twitter.com/WhatDaMath.
Older adults who sleep just five hours a night or fewer have a greater risk of developing more than one chronic disease, new research shows. The findings underscore the importance of healthy sleep patterns throughout life, and especially in middle and old age.
The new study, published in PLOS Medicine, examined sleep duration and its effect on multimorbidity—or the occurrence of more than one chronic condition, like diabetes and cardiovascular disease, at once. People ages 50 or older who slept a total of five hours a night or less were found to have at least a 30% greater risk of multimorbidity.
Prior studies have largely focused on the link between sleep and the development of individual chronic diseases, but it’s been unclear how sleep duration contributes to the development of multiple chronic conditions. The new findings add to growing evidence suggesting that sleep deficiencies can affect health outcomes.
It gives new meaning to the phrase “speak your mind.
Do you remember how legendary cosmologist Stephen Hawking communicated using his special screen-equipped chair? Well, that was a brain-computer interface (BCI), a device that allows a person to communicate using their brain signals.
There are approximately 70 million people across the globe who suffer from speech-related disorders. What if there was a BCI for each one of these patients that could at least spell out words, if not speak for them? A team of researchers from the University of California, San Fransico (UCSF) works on one such groundbreaking device.
They have created a neuroprosthesis (a type of BCI device that re-establish lost functions of the nervous system) that analyzes the brain activity of a user with speech paralysis. The device then translates the brain signals into single letters and spells sentences on a screen. Reading the sentences lets anyone know what the user wants to say.