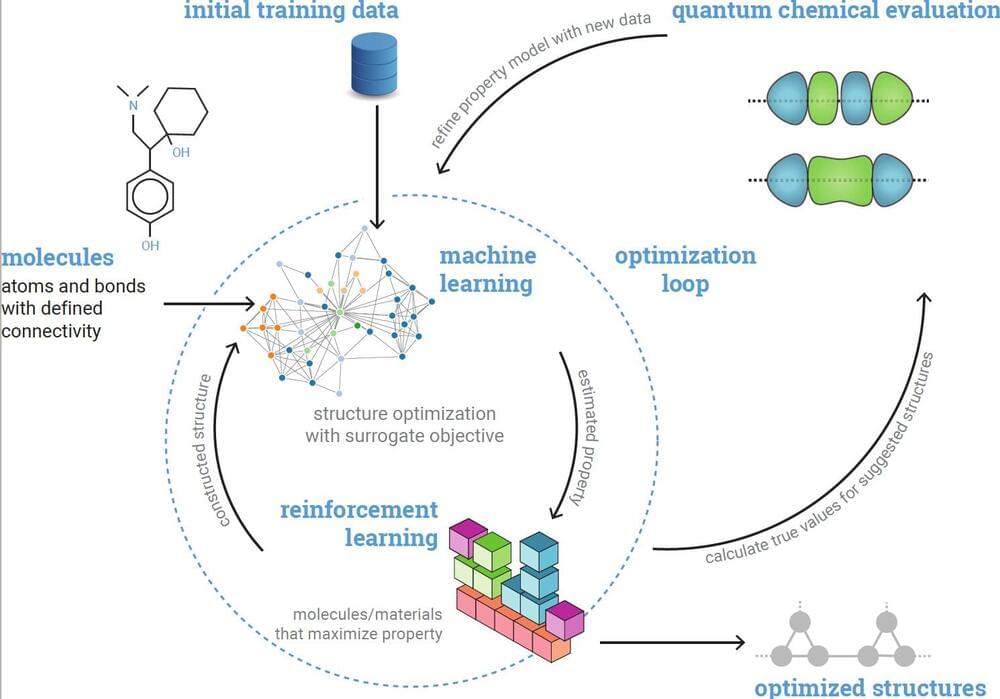
Recent advancements in the development of machine learning and optimization techniques have opened new and exciting possibilities for identifying suitable molecular designs, compounds, and chemical candidates for different applications. Optimization techniques, some of which are based on machine learning algorithms, are powerful tools that can be used to select optimal solutions for a given problem among a typically large set of possibilities.
Researchers at Colorado State University and the National Renewable Energy Laboratory have been applying state-of-the-art molecular optimization models to different real-world problems that entail identifying new and promising molecular designs. In their most recent study, featured in Nature Machine Intelligence, they specifically applied a newly developed, open-source optimization framework to the task of identifying viable organic radicals for aqueous redox flow batteries, energy devices that convert chemical energy into electricity.
“Our project was funded by an ARPA-E program that was looking to shorten how long it takes to develop new energy materials using machine learning techniques,” Peter C. St. John, one of the researchers who carried out the study, told TechXplore. “Finding new candidates for redox flow batteries was an interesting extension of some of our previous work, including a paper published in Nature Communications and another in Scientific Data, both looking at organic radicals.”