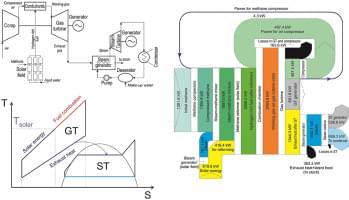
A transition to a carbon-free economy is the reality of the modern energy industry. Reduction in CO2 emission is one of the main challenge in energy engineering in the last decades. Renewable energy sources are playing an important role on the way to a zero-carbon economy [1,2]. Solar energy is one of the main and almost unlimited energy sources in the World. The different technologies of solar energy use have been developed in the last years [[3], [4], [5], [6], [7], [8]]. However, even though the progress in the development of solar energy technologies is notable, there are a lot of challenges for energy science. One of them is the fact that more than 60% of electricity is produced by conventional technologies via hydrocarbon fuel combustion: steam turbines, gas turbines, etc. While the share of electricity produced by using solar energy is no more than a few percent [9].
Among various ways of utilization of solar energy for electricity generation, a combination of solar energy with the traditional steam and gas turbine cycles can be highlighted. The power plants where solar energy is combined with conventional power cycles are named integrated solar combined cycle systems (ISCCS). In these systems, solar energy is used to produce heat and after that heat is used to generate mechanical work or electricity.
Combined cycle power plants (CCPP) show one of the highest energy efficiency among conventional power plants [10]. The modern cycles with high-temperature gas turbines have an efficiency up to 70% and even higher. In such cycles, the high-temperature gas turbines with the turbine inlet temperature (TIT) up to 1,600 °C are applied [11,12]. In the last years, a lot of various integrated solar combined cycle systems (ISCCS) were developed by various scientists and engineers. The main way to use solar energy in such cycles is a steam generation in CCPP [[13], [14], [15], [16]]. In other words, solar energy in such ISCCS is utilized as an energy source in a steam turbine cycle.