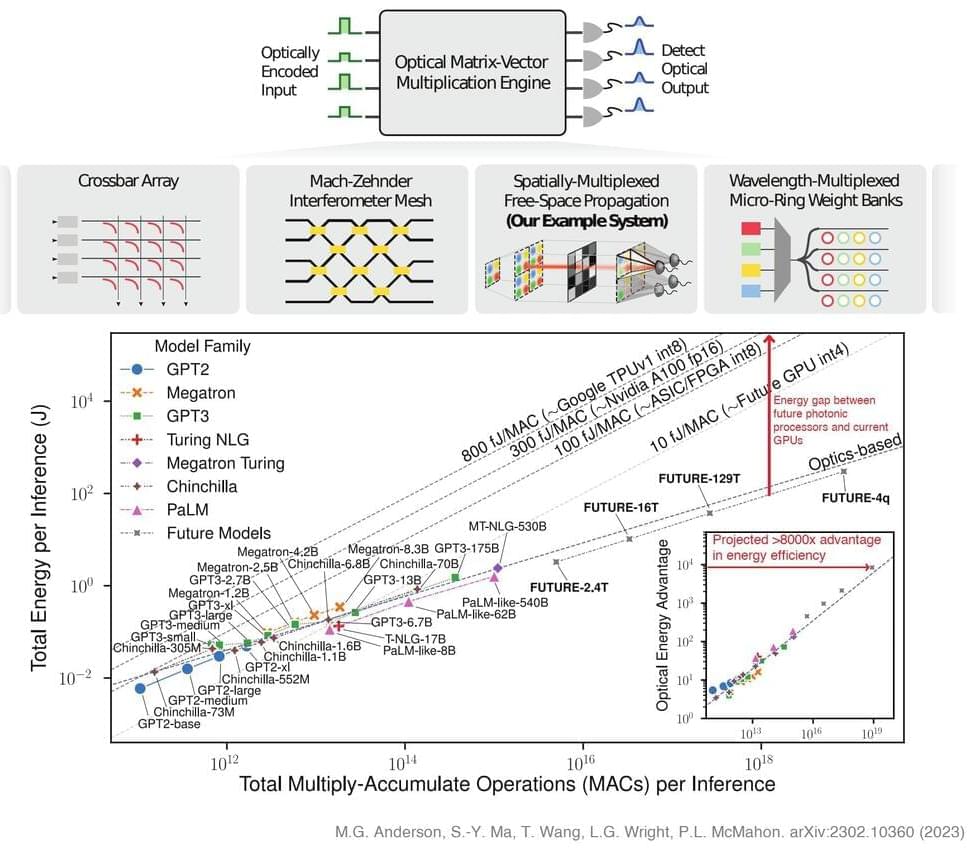
The exponentially expanding scale of deep learning models is a major force in advancing the state-of-the-art and a source of growing worry over the energy consumption, speed, and, therefore, feasibility of massive-scale deep learning. Recently, researchers from Cornell talked about Transformer topologies, particularly how they are dramatically better when scaled up to billions or even trillions of parameters, leading to an exponential rise in the utilization of deep learning computing. These large-scale Transformers are a popular but expensive solution for many tasks because digital hardware’s energy efficiency has not kept up with the rising FLOP requirements of cutting-edge deep learning models. They also perform increasingly impressively in other domains, such as computer vision, graphs, and multi-modal settings.
Also, they exhibit transfer learning skills, which enable them to quickly generalize to certain activities, sometimes in a zero-shot environment with no additional training required. The cost of these models and their general machine-learning capabilities are major driving forces behind the creation of hardware accelerators for effective and quick inference. Deep learning hardware has previously been extensively developed in digital electronics, including GPUs, mobile accelerator chips, FPGAs, and large-scale AI-dedicated accelerator systems. Optical neural networks have been suggested as solutions that provide better efficiency and latency than neural-network implementations on digital computers, among other ways. At the same time, there is also significant interest in analog computing.
Even though these analog systems are susceptible to noise and error, neural network operations can frequently be carried out optically for a much lower cost, with the main cost typically being the electrical overhead associated with loading the weights and data amortized in large linear operations. The acceleration of huge-scale models like Transformers is thus particularly promising. Theoretically, the scaling is asymptotically more efficient regarding energy per MAC than digital systems. Here, they demonstrate how Transformers use this scaling more and more. They sampled operations from a real Transformer for language modeling to run on a real spatial light modulator-based experimental system. They then used the results to create a calibrated simulation of a full Transformer running optically. This was done to show that Transformers may run on these systems despite their noise and error characteristics.