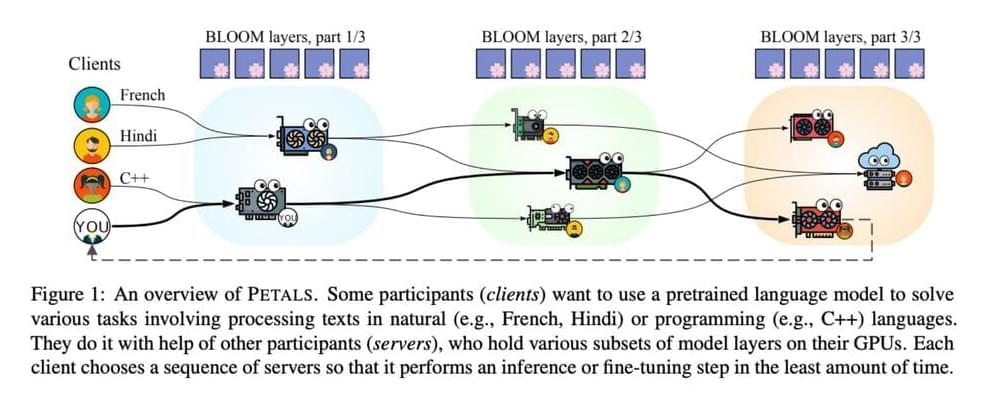
The NLP community has recently discovered that pretrained language models may accomplish various real-world activities with the help of minor adjustments or direct assistance. Additionally, performance usually becomes better as the size grows. Modern language models often include hundreds of billions of parameters, continuing this trend. Several research groups published pretrained LLMs with more than 100B parameters. The BigScience project most recently made BLOOM available, a 176 billion parameter model that supports 46 natural and 13 computer languages. The public availability of 100B+ parameter models makes them more accessible, yet due to memory and computational expenses, most academics and practitioners still find it challenging to use them. For inference, OPT-175B and BLOOM-176B require more than 350GB of accelerator RAM and even more for finetuning.
As a result, running these LLMs typically requires several powerful GPUs or multi-node clusters. These two alternatives are relatively inexpensive, restricting the potential study topics and language model applications. By “offloading” model parameters to slower but more affordable memory and executing them on the accelerator layer by layer, several recent efforts seek to democratize LLMs. By loading parameters from RAM just in time for each forward pass, this technique enables executing LLMs with a single low-end accelerator. Although offloading has high latency, it can process several tokens in parallel. For instance, they are producing one token with BLOOM-176B requires at least 5.5 seconds for the fastest RAM offloading system and 22 seconds for the quickest SSD offloading arrangement.
Additionally, many machines lack sufficient RAM to unload 175B parameters. LLMs may be made more widely available through public inference APIs, where one party hosts the model and allows others to query it online. This is a fairly user-friendly choice because the API owner handles most of the engineering effort. However, APIs are frequently too rigid to be used in research since they cannot alter the model’s control structure or have access to its internal states. Additionally, the cost of some research initiatives may be exorbitant, given the current API price. In this study, they investigate a different approach motivated by widespread crowdsourcing training of neural networks from scratch.