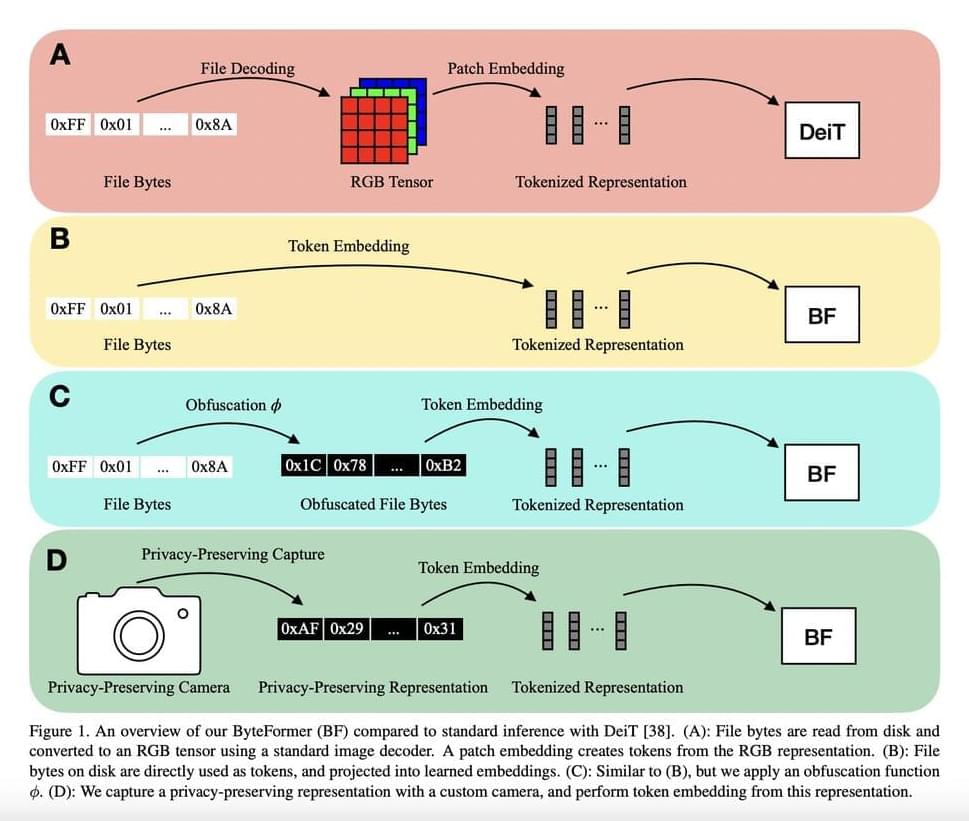
The explicit modeling of the input modality is typically required for deep learning inference. For instance, by encoding picture patches into vectors, Vision Transformers (ViTs) directly model the 2D spatial organization of images. Similarly, calculating spectral characteristics (like MFCCs) to transmit into a network is frequently involved in audio inference. A user must first decode a file into a modality-specific representation (such as an RGB tensor or MFCCs) before making an inference on a file that is saved on a disc (such as a JPEG image file or an MP3 audio file), as shown in Figure 1a. There are two real downsides to decoding inputs into a modality-specific representation.
It first involves manually creating an input representation and a model stem for each input modality. Recent projects like PerceiverIO and UnifiedIO have demonstrated the versatility of Transformer backbones. These techniques still need modality-specific input preprocessing, though. For instance, before sending picture files into the network, PerceiverIO decodes them into tensors. Other input modalities are transformed into various forms by PerceiverIO. They postulate that executing inference directly on file bytes makes it feasible to eliminate all modality-specific input preprocessing. The exposure of the material being analyzed is the second disadvantage of decoding inputs into a modality-specific representation.
Think of a smart home gadget that uses RGB photos to conduct inference. The user’s privacy may be jeopardized if an enemy gains access to this model input. They contend that deduction can instead be carried out on inputs that protect privacy. They make notice that numerous input modalities share the ability to be saved as file bytes to solve these shortcomings. As a result, they feed file bytes into their model at inference time (Figure 1b) without doing any decoding. Given their capability to handle a range of modalities and variable-length inputs, they adopt a modified Transformer architecture for their model.