Or is it playing a different game than the US?
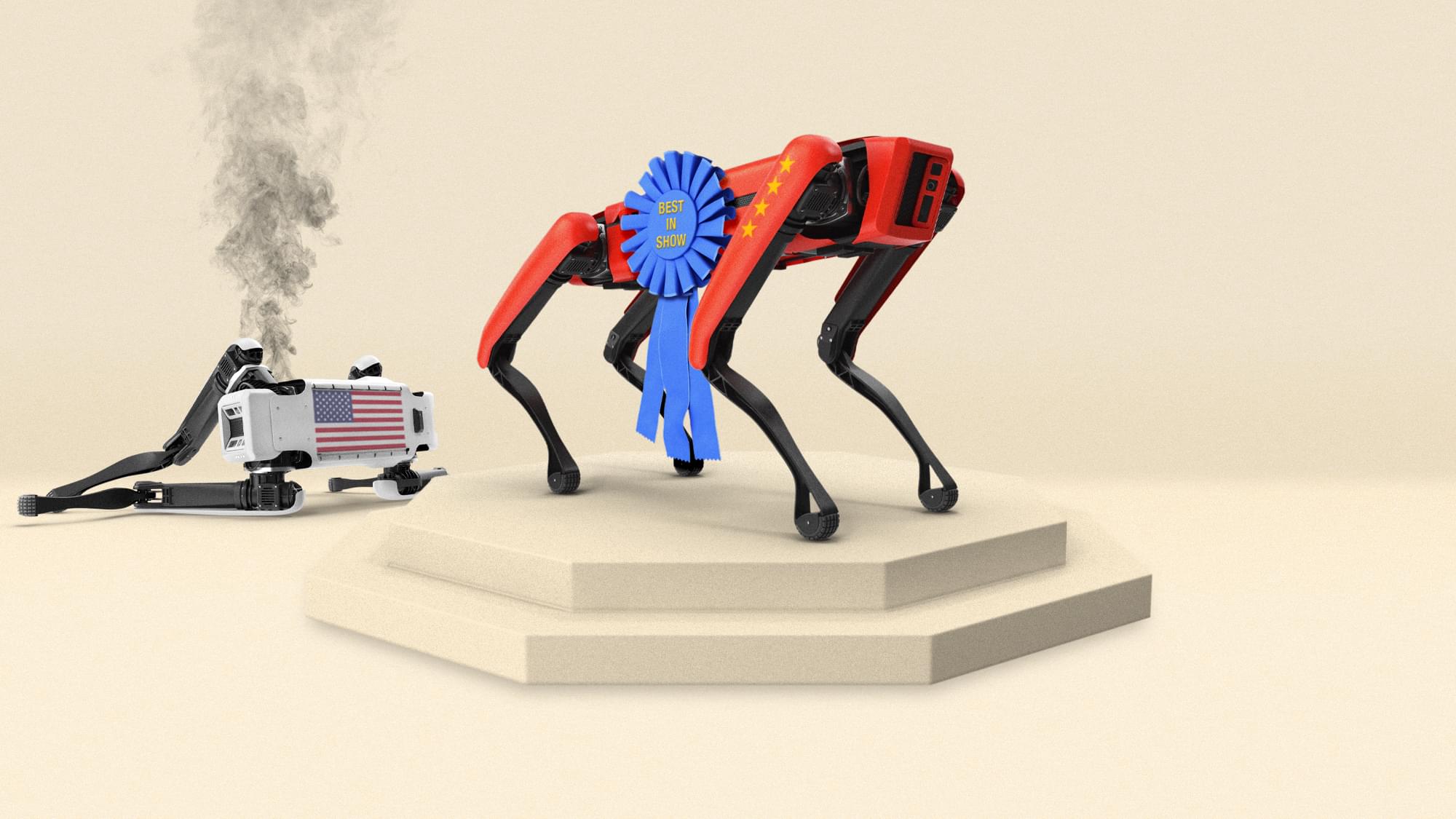
In the intriguing realm of star-forming galaxies, the key factor isn’t the total amount of gas but rather its strategic distribution within the galaxy.
Researchers at the International Centre for Radio Astronomy Research (ICRAR) made the discovery about galaxies by studying the gas distribution that helps create stars.
Using CSIRO’s ASKAP radio telescope located at Inyarrimanha Ilgari Bundara, the CSIRO Murchison Radio-astronomy Observatory, researchers explored the gas distribution in about 1,000 galaxies as part of the WALLABY survey.
A University of Nebraska–Lincoln engineering team is another step closer to developing soft robotics and wearable systems that mimic the ability of human and plant skin to detect and self-heal injuries.
Husker engineer Eric Markvicka, along with graduate students Ethan Krings and Patrick McManigal, recently presented a paper at the prestigious IEEE International Conference on Robotics and Automation in Atlanta, Georgia, that sets forth a systems-level approach for a soft robotics technology that can identify damage from a puncture or extreme pressure, pinpoint its location and autonomously initiate self-repair.
The paper was among the 39 of 1,606 submissions selected as an ICRA 2025 Best Paper Award finalist. It was also a finalist for the Best Student Paper Award and in the mechanism and design category.
#Repost
A Husker engineering team is another step closer to developing soft robotics and wearable systems that mimic the ability of human and plant skin to detect and self-heal injuries.
The Vera C. Rubin Observatory in Chile will image the southern sky using the largest digital camera ever built.
Among the crystalline materials whose edges host exotic quantum states are the Dirac semimetal and the Chern insulator. In the semimetal, the edge states are localized and not protected from perturbations by their topology; in the insulator, they move in one direction and are protected. Proposed by theorists in 2011, a hybrid phase called a Chern semimetal simultaneously hosts localized and one-way edge states, both with topological protection (see Synopsis: Quantum Hall Anomaly in 3D). Now Jianfeng Chen and Cheng-Wei Qiu at the National University of Singapore and their colleagues have realized this phase in a two-dimensional photonic metamaterial [1]. The researchers say that their system provides a platform for nanophotonic applications and a playground for topological physics.
The researchers built a honeycomb-shaped, single-layer array of millimeter-sized magnetic rods. They then applied a carefully tuned, spatially varying magnetic field to this array, creating a pattern in which only specific rods were magnetized. Lastly, they placed a source of microwaves at the array’s boundary and observed how the microwave radiation traveled through the structure. The team found that the magnetization pattern caused some radiation to localize at the array’s boundary and other radiation to move in one direction around the boundary. All these edge states were topologically protected in that they were immune to scattering caused by slight differences in the alignment, shape, and size of the rods and other material imperfections.
Thanks to the coexistence and potential hybridization of localized and one-way states, a photonic Chern semimetal can reduce the speed of transmitted radiation. The researchers found that their metamaterial exhibited a slowdown factor of up to 30 while preserving the radiation’s topological protection. They say that this capability could be useful for nanophotonic technology.
Adatoms are single atoms that get adsorbed onto the surface of a solid material and are known to hop randomly from one spot to another. In a recent study published in Nature Communications, a group of scientists from Germany demonstrated that single atoms can be steered in a chosen direction at near absolute zero temperatures (4 Kelvin), provided the surface being used is magnetic in nature—a discovery that can open up new possibilities for precise control of atomic motion, a sought-after ability in the field of nanotechnology, data storage and functional materials.
The researchers placed individual cobalt, rhodium, and iridium atoms on a 1-atom-thick manganese surface to create a magnetically well-defined surface and studied the migration behavior of adatoms using a scanning tunneling microscope (STM) at a temperature of 4 K.
According to established findings from nonmagnetic surfaces, atomic movement is usually governed by surface symmetry. In a hexagonal manganese monolayer like the one used in the study, atoms would be expected to migrate randomly in any of six directions. Yet in a surprising twist, researchers found that when a short, localized voltage pulse from the STM was applied, the atoms consistently moved in just one direction.
Using the Low Frequency Array (LOFAR), European astronomers have investigated a galaxy cluster designated CIZA J2242.8+5301, dubbed the Sausage cluster. The observations conducted at very low radio frequencies provide more insights into the properties of radio relics in this cluster. The new findings are presented in a research paper published May 29 on the arXiv preprint server.
Galaxy clusters consist of up to thousands of galaxies bound together by gravity. They are the largest known gravitationally-bound structures in the universe, and therefore serve as excellent laboratories for studying galaxy evolution and cosmology. Observations show that galaxy clusters generally form as a result of mergers and grow by accreting sub-clusters.
CIZA J2242.8+5301 is a well-studied merging galaxy cluster at a redshift of 0.192. It contains prominent double radio relics (diffuse, elongated radio sources of synchrotron origin) and other diffuse radio sources. CIZA J2242.8+5301 was nicknamed the Sausage cluster due to the distinctive morphology of its northern relic.