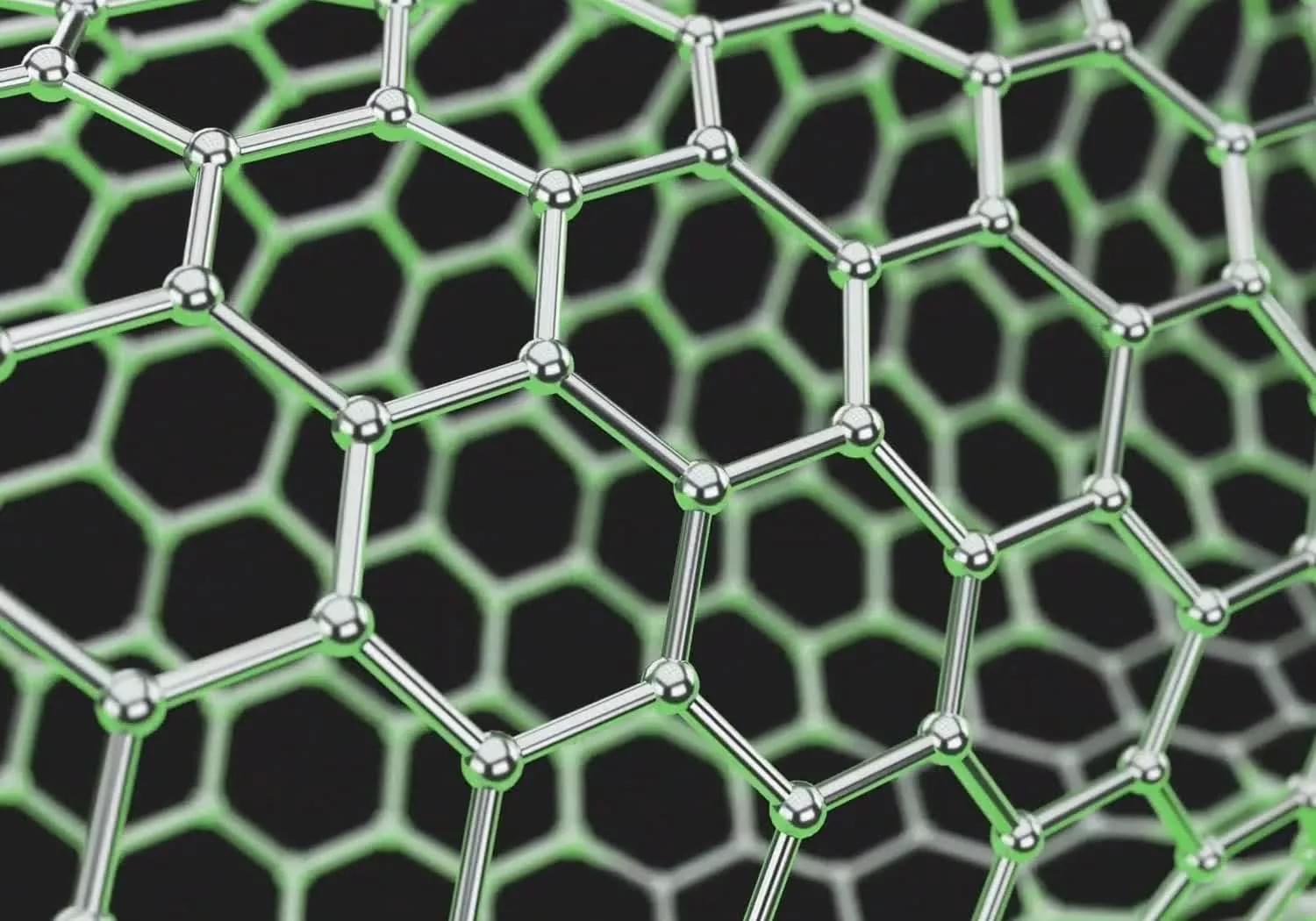
The research team, led by Professor Tobin Filleter, has engineered nanomaterials that offer unprecedented strength, weight, and customizability. These materials are composed of tiny building blocks, or repeating units, measuring just a few hundred nanometers – so small that over 100 lined up would barely match the thickness of a human hair.
The researchers used a multi-objective Bayesian optimization machine learning algorithm to predict optimal geometries for enhancing stress distribution and improving the strength-to-weight ratio of nano-architected designs. The algorithm only needed 400 data points, whereas others might need 20,000 or more, allowing the researchers to work with a smaller, high-quality data set. The Canadian team collaborated with Professor Seunghwa Ryu and PhD student Jinwook Yeo at the Korean Advanced Institute of Science & Technology for this step of the process.
This experiment was the first time scientists have applied machine learning to optimize nano-architected materials. According to Peter Serles, the lead author of the project’s paper published in Advanced Materials, the team was shocked by the improvements. It didn’t just replicate successful geometries from the training data; it learned from what changes to the shapes worked and what didn’t, enabling it to predict entirely new lattice geometries.