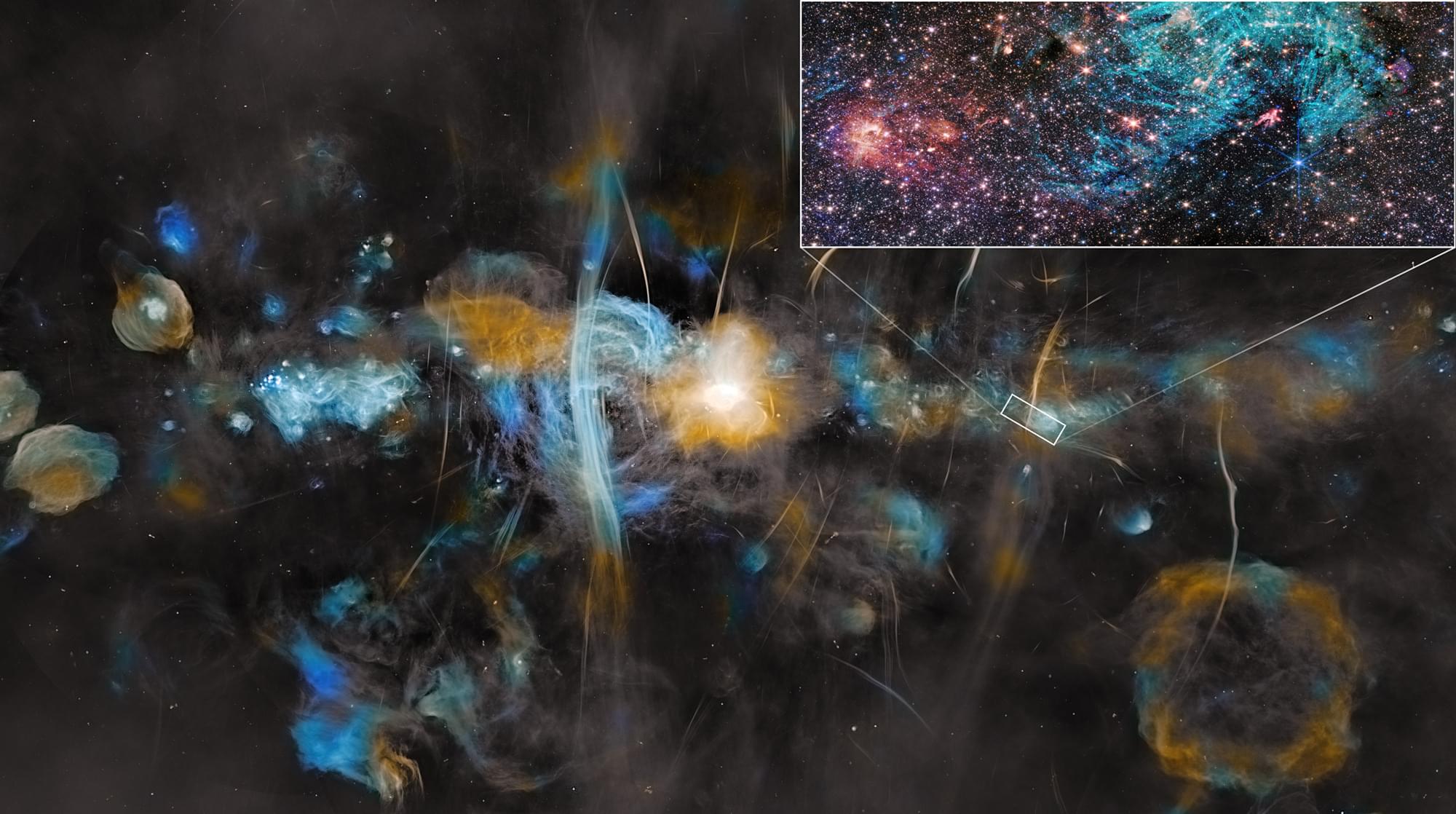
Follow-up research on a 2023 image of the Sagittarius C stellar nursery in the heart of our Milky Way galaxy, captured by NASA’s James Webb Space Telescope, has revealed ejections from still-forming protostars and insights into the impact of strong magnetic fields on interstellar gas and the life cycle of stars.
“A big question in the Central Molecular Zone of our galaxy has been, if there is so much dense gas and cosmic dust here, and we know that stars form in such clouds, why are so few stars born here?” said astrophysicist John Bally of the University of Colorado Boulder, one of the principal investigators. “Now, for the first time, we are seeing directly that strong magnetic fields may play an important role in suppressing star formation, even at small scales.”
Detailed study of stars in this crowded, dusty region has been limited, but Webb’s advanced near-infrared instruments have allowed astronomers to see through the clouds to study young stars like never before.