We hope you bought an SSD last year when prices were affordable.
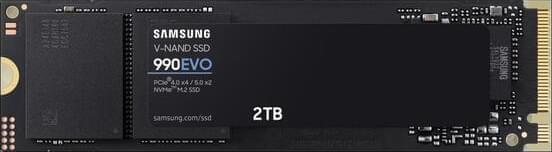
The next-gen Chevy Bolt EV is due out next year after GM ended production of its top-selling electric model at the end of 2023. GM’s CFO Paul Jacobson says the new Bolt EV will save the company billions by becoming North America’s first Ultium-based model to use LFP batteries.
After EV enthusiasts like myself were disappointed to learn GM planned to discontinue the electric Chevy Bolt EV last year, GM’s CEO Mary Barra revealed a next-gen model was in the works.
During a media call last July, Barra revealed the Bolt EV will live on as an Ultium-based model, claiming it will streamline production.
Webb telescope observes potentially young Methane deposits on surfaces of Eris, Makemake. A team co-led by Southwest Research Institute found evidence for hydrothermal or metamorphic activity within the icy dwarf planets Eris and Makemake, located in the Kuiper Belt. Methane detected on their surfaces has the tell-tale signs of warm or even hot geochemistry in their rocky cores, which is markedly different than the signature of methane from a comet.
“We see some interesting signs of hot times in cool places,” said SwRI’s Dr. Christopher Glein, an expert in planetary geochemistry and lead author of a paper about this discovery.
The Kuiper Belt is a vast donut-shaped region of icy bodies beyond the orbit of Neptune at the edge of the solar system.
NASA’s James Webb Space Telescope (JWST) has spotted a rare and “extremely red” supermassive black hole lurking in one of the most ancient corners of the universe.
Astronomers suggest the vermilion black hole was the result of an expanding universe just 700 million years following the Big Bang, as detailed in a paper published this month in the journal Nature. Its colors are likely due to a thick layer of dust blocking much of its light, they posit.
While the cosmic monster was technically first discovered last year, researchers have now found that it’s far more massive than any other object of its kind in the area, making it a highly unusual find that could rewrite the way we understand how supermassive black holes grow relative to their host galaxies.
A team of researchers have claimed to have recently detected a telltale signature of stimulated Hawking radiation from a post-merger black hole. If the researchers’ analysis of gravitational wave data is correct, then they may have found the first evidence of Planck-scale quantum structure at the event horizon of a black hole (quantum horizons). The key signature of a non-classical horizon is an echo signal in the gravitational waves that are detected after the primary merger event of a binary black hole system. The evidence is tentative, but nevertheless tantalizing. Such research is pivotal to advancing our understanding of quantum effects in strong gravity, where novel aspects of the theory of quantum gravity may be hard at work, as exemplified in the remarkable research The Origin of Mass and the Nature of Gravity, in which physicist Nassim Haramein with his colleagues Dr. Olivier Alirol and Dr. Cyprien Guermonprez have demonstrated that the mass-energy of Hawking radiation from a baryonic-scale mini black hole exactly produces the observed rest-mass energy of the proton, demonstrating that the proton rest-mass is the result of quantum vacuum fluctuations of the electromagnetic field in strongly curved spacetime. The analysis of gravitational wave data for an echo signature, the smoking gun of quantum horizons and Hawking radiation, in conjunction with recent observation of Unruh radiation from accelerating electrons, is a significant confirmation of quantum gravitational predictions of unified physics, which we see in solutions like that of Haramein et al. are the solution to understanding the source of mass and force originating from quantum vacuum fluctuations in curved spacetime. It is a major advancement because Unruh-Hawking radiation can no longer be said to be “only theoretical”