This breakthrough in plasma stability brings the dream of endless energy even closer.
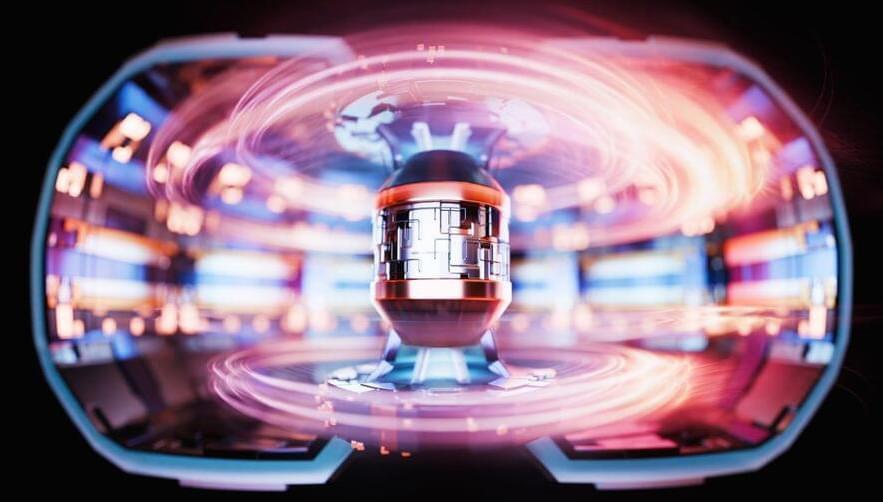
Neuralink’s first human patient has become so adept at using the company’s brain implant that he can now beat other players at video games.
On Wednesday, Elon Musk’s company provided a progress update on Noland Arbaugh, who received a brain implant in January that lets him remotely control the cursor on a laptop.
In March, Neuralink revealed that Arbaugh was using the implant to play games including Chess, Civilization VI, and Mario Kart. In Wednesday’s update, the company reported that Arbaugh’s use of the implant has only improved over time.
Does free will exist? Neil deGrasse Tyson and Chuck Nice sit down with astrophysicist Charles Liu sit down to discuss the existence of free will and whether physics allows for choice in our lives.
We explore cause and effect: how does uncertainty and chaos in the universe factor into free will? How important is the illusion of free will to society? What does a society that acknowledges a lack of free will look like?
Check out our second channel, @StarTalkPlus.
Get the NEW StarTalk book, ‘To Infinity and Beyond: A Journey of Cosmic Discovery’ on Amazon: https://amzn.to/3PL0NFn.
Support us on Patreon: / startalkradio.
FOLLOW or SUBSCRIBE to StarTalk:
“There is still a great deal of stigma around the use of substances during pregnancy,” said Dr. Jamie Lo, M.D., M.C.R. “Our hope is that this research supports more open and productive conversations that ultimately result in a healthier pregnancy.”
It has long been known that smoking during pregnancy can result in bad health for newborns, but what are the consequences of smoking both nicotine and cannabis during pregnancy? This is what a recent study published in JAMA hopes to address as a team of researchers investigated the potential health risks for newborns when pregnant mothers smoke both nicotine and cannabis during pregnancy. This study holds the potential to help researchers, medical practitioners, and the public better understand the health risks of cannabis as its recreational use continues to become legalized across the United States.
“With the growing legalization of cannabis around the country, there is often a perception that cannabis is safe in pregnancy,” said Dr. Jamie Lo, M.D., M.C.R., who is an associate professor of obstetrics and gynecology at the Oregon Health & Science University School of Medicine and a co-author on the study. “Because we know that many people who use cannabis often use tobacco or nicotine products, we wanted to better understand the potential health implications on both the pregnant individual and the infant.
For the study, the researchers analyzed hospital discharge data of 3,129,259 pregnant women whose records were obtained from the California Department of Public Health and the California Department of Health Care Access and Information with the goal of using specific health codes to ascertain cannabis and nicotine use during pregnancy, and specifically the health outcomes of their newborns resulting from this exposure. In the end, the researchers determined that 23,007 used cannabis during pregnancy, 56,811 used nicotine during pregnancy, and 10,312 used both during pregnancy.
Save the date for Free the Pill’s 6th annual Free the Pill Day on May 9—the same day that the first birth control pill was approved by the US FDA back in 1960!
This year’s Free the Pill Day will be the first one with an OTC birth control pill on the shelf! On May 9, we invite you to join us on social media throughout the day to celebrate Opill on the shelf, spread evidence-based information about Opill and how to get it, and highlight the need for continued work to support equitable access—including advocating for full insurance coverage, low and no-cost options, and availability in stores and online without barriers.