OpenAI has delayed its Voice Mode feature that allows users to have near-real-time conversations with ChatGPT.
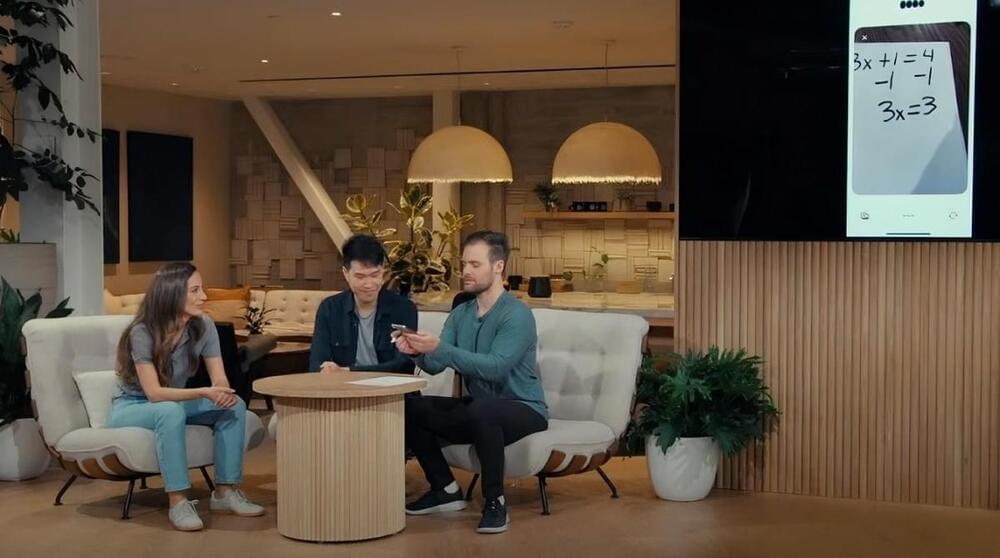
Researchers propose a neuron-as-controller model, challenging the simplistic 1960s-era computational model of neurons.
Researchers at the University of Vienna led by Philip Walther just pioneered the field of quantum mechanics and general relativity by measuring “the effect of the rotation of Earth on quantum entangled photons,” as stated in a press release.
In the Vienna experiment, they used an interferometer, which is the most sensitive to rotations. Its unparalleled precision makes it the ultimate tool for measuring rotational speeds, limited only by the boundaries of classical physics.
The team wondered if they could somehow leverage crystalline structures to identify a perfect candidate, sans building thousands of them in a lab.
The researchers were mostly on the lookout for 3D crystals with the right structural and electronic properties, so they could be “exfoliated.” 2D materials like graphene were extracted using this process from 3D.
However, this would be the first time researchers exfoliated one-dimensional materials like carbon nanotubes. This approach created a database of around 78,000 known 3D crystalline structures.
MIT just produced three groundbreaking innovations that allowed them to map whole hemispheres of the human brain.
In a world first, a team of scientists has successfully developed the first flexible perovskite/silicon tandem solar cell with a record efficiency of 22.8 percent.
While other scientists have developed flexible solar cells before, the new efficiency record sets a new precedent and represents a big step forward for the technology.
It shows that flexible perovskite/silicon tandem solar cells are feasible, meaning they could soon be used for a large variety of applications.
GXO has partnered with Apptronik to explore Apollo humanoid robot’s use in warehouses. Apollo is the first commercial humanoid robot that was designed for friendly interaction and mass manufacturability.
SpaceX has introduced a compact version of satellite internet antennas. Called Starlink Mini, the antenna is portable and can be packed in a backpack. The product is dubbed revolutionary due to being a mobile option for satellite internet customers.
Currently, a limited number of antennas are being offered for just $599 each in an early access release. Starlink Mini integrates the WiFi router right inside the dish and can deliver over 100mbps speed.
Elon Musk claims the product has the power to change the world.
A recent study published in the journal Artificial Intelligence in Geosciences introduced an advanced method for automatic microfossil detection and analysis. The research team consisted of members from the machine learning group at the University of Tromso (UiT) The Arctic University of Norway.
They have developed a pipeline for extracting fossil information from microscope slide images. They found that deep learning techniques outperform traditional image processing methods and that self-supervision can be effectively used for feature extraction.