Astronomers anticipate an unprecedented view of the solar system as the Rubin Observatory begins to scan millions of hidden celestial objects.
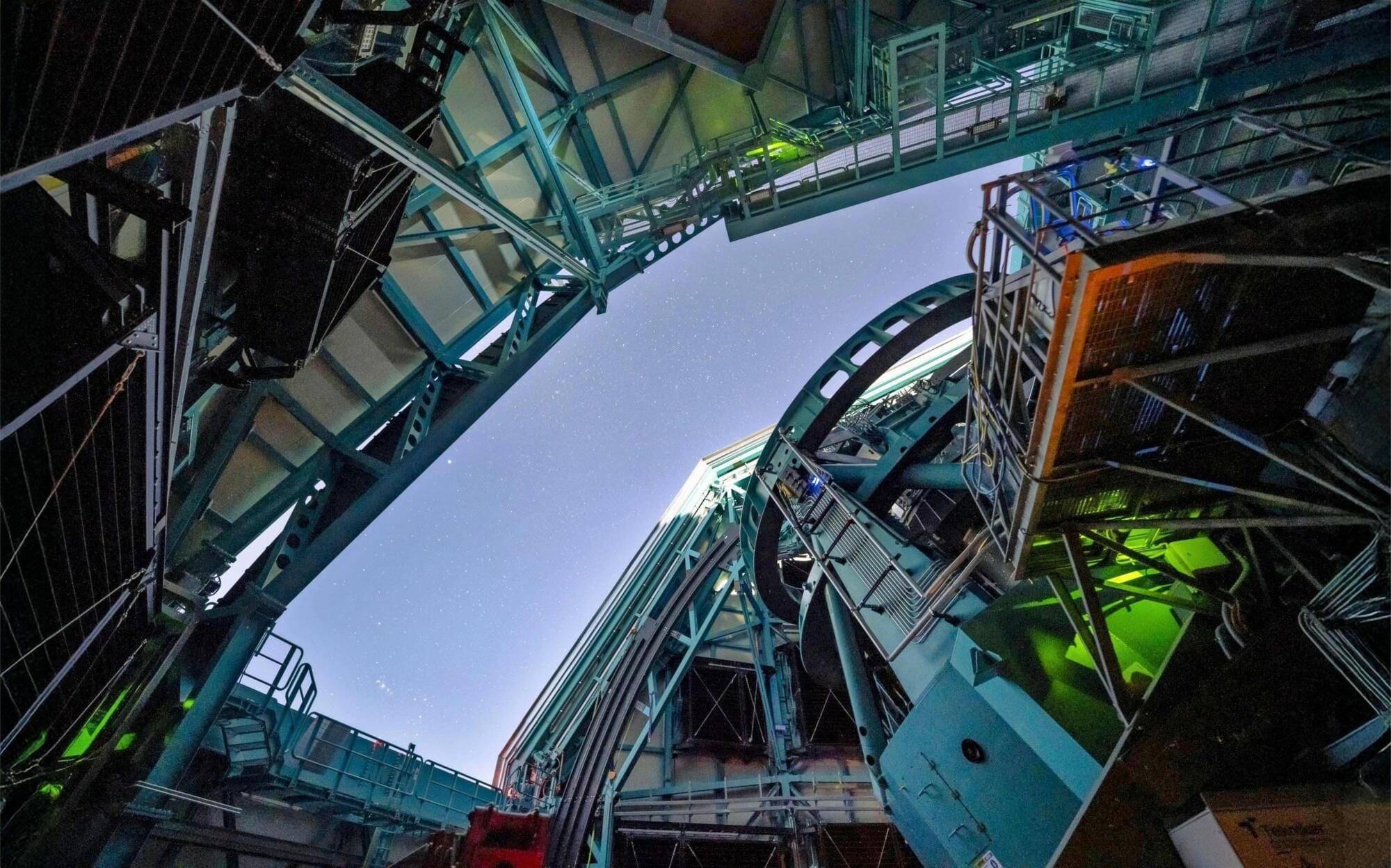
The entire field of mathematics summarised in a single map! This shows how pure mathematics and applied mathematics relate to each other and all of the sub-topics they are made from.
#mathematics #DomainOfScience.
If you would like to buy a poster of this map, they are available here:
North America: https://store.dftba.com/products/map–… else: http://www.redbubble.com/people/domin… French version: https://www.redbubble.com/people/domi… Spanish Version: https://www.redbubble.com/people/domi… I have also made a version available for educational use which you can find here: https://www.flickr.com/photos/9586967… To err is to human, and I human a lot. I always try my best to be as correct as possible, but unfortunately I make mistakes. This is the errata where I correct my silly mistakes. My goal is to one day do a video with no errors! 1. The number one is not a prime number. The definition of a prime number is a number can be divided evenly only by 1, or itself. And it must be a whole number GREATER than 1. (This last bit is the bit I forgot). 2. In the trigonometry section I drew cos(theta) = opposite / adjacent. This is the kind of thing you learn in high school and guess what. I got it wrong! Dummy. It should be cos(theta) = adjacent / hypotenuse. 3. My drawing of dice is slightly wrong. Most dice have their opposite sides adding up to 7, so when I drew 3 and 4 next to each other that is incorrect. 4. I said that the Gödel Incompleteness Theorems implied that mathematics is made up by humans, but that is wrong, just ignore that statement. I have learned more about it now, here is a good video explaining it: • Gödel’s Incompleteness Theorem — Numberphile 5. In the animation about imaginary numbers I drew the real axis as vertical and the imaginary axis as horizontal which is opposite to the conventional way it is done. Thanks so much to my supporters on Patreon. I hope to make money from my videos one day, but I’m not there yet! If you enjoy my videos and would like to help me make more this is the best way and I appreciate it very much.
/ domainofscience Here are links to some of the sources I used in this video. Links: Summary of mathematics: https://en.wikipedia.org/wiki/Mathema… Earliest human counting: http://mathtimeline.weebly.com/early–… First use of zero: https://en.wikipedia.org/wiki/0#History http://www.livescience.com/27853-who–… First use of negative numbers: https://www.quora.com/Who-is-the-inve… Renaissance science: https://en.wikipedia.org/wiki/History… History of complex numbers: http://rossroessler.tripod.com/ https://en.wikipedia.org/wiki/Mathema… Proof that pi is irrational: https://www.quora.com/How-do-you-prov… and https://en.wikipedia.org/wiki/Proof_t… Also, if you enjoyed this video, you will probably like my science books, available in all good books shops around the work and is printed in 16 languages. Links are below or just search for Professor Astro Cat. They are fun children’s books aimed at the age range 7–12. But they are also a hit with adults who want good explanations of science. The books have won awards and the app won a Webby. Frontiers of Space: http://nobrow.net/shop/professor-astr… Atomic Adventure: http://nobrow.net/shop/professor-astr… Intergalactic Activity Book: http://nobrow.net/shop/professor-astr… Solar System App: Find me on twitter, instagram, and my website: http://dominicwalliman.com
/ dominicwalliman
/ dominicwalliman
/ dominicwalliman.
Everywhere else: http://www.redbubble.com/people/domin…
French version: https://www.redbubble.com/people/domi…
Spanish Version: https://www.redbubble.com/people/domi…
I have also made a version available for educational use which you can find here: https://www.flickr.com/photos/9586967…
To err is to human, and I human a lot. I always try my best to be as correct as possible, but unfortunately I make mistakes. This is the errata where I correct my silly mistakes. My goal is to one day do a video with no errors!
1. The number one is not a prime number. The definition of a prime number is a number can be divided evenly only by 1, or itself. And it must be a whole number GREATER than 1. (This last bit is the bit I forgot).
A team of chemists at the University of Cambridge has developed a powerful new method for adding single carbon atoms to molecules more easily, offering a simple one-step approach that could accelerate drug discovery and the design of complex chemical products.
The research, recently published in the journal Nature under the title “One-carbon homologation of alkenes,” unveils a breakthrough method for extending molecular chains—one carbon atom at a time. This technique targets alkenes, a common class of molecules characterized by a double bond between two carbon atoms. Alkenes are found in a wide range of everyday products, from anti-malarial medicines like quinine to agrochemicals and fragrances.
Led by Dr. Marcus Grocott and Professor Matthew Gaunt from the Yusuf Hamied Department of Chemistry at the University of Cambridge, the work replaces traditional multi-step procedures with a single-pot reaction that is compatible with a wide range of molecules.
The reliable manipulation of the speed at which light travels through objects could have valuable implications for the development of various advanced technologies, including high-speed communication systems and quantum information processing devices. Conventional methods for manipulating the speed of light, such as techniques leveraging so-called electromagnetically induced transparency (EIT) effects, work by utilizing quantum interference effects in a medium, which can make it transparent to light beams and slow the speed of light through it.
Despite their advantages, these techniques only enable the reciprocal control of group velocity (i.e., the speed at which the envelope of a wave packet travels through a medium), meaning that a light beam will behave the same irrespective of the direction it is traveling in while passing through a device. Yet the nonreciprocal control of light speed could be equally valuable, particularly for the development of advanced devices that can benefit from allowing signals to travel in desired directions at the desired speed.
Researchers at the University of Manitoba in Canada and Lanzhou University in China recently demonstrated the nonreciprocal control of the speed of light using a cavity magnonics device, a system that couples microwave photons (i.e., quanta of microwave light) with magnons (i.e., quanta of the oscillations of electron spins in materials).
Pressure is on Apple to show it hasn’t lost its magic despite broken promises to ramp up iPhones with generative artificial intelligence (GenAI) as rivals race ahead with the technology.
Apple will showcase plans for its coveted devices and the software powering them at its annual Worldwide Developers Conference (WWDC) kicking off Monday in Silicon Valley.
The event comes a year after the tech titan said a suite of AI features it dubbed “Apple Intelligence” was heading for iPhones, including an improvement of its much criticized Siri voice assistant.
Whether you’re streaming a show, paying bills online or sending an email, each of these actions relies on computer programs that run behind the scenes. The process of writing computer programs is known as coding. Until recently, most computer code was written, at least originally, by human beings. But with the advent of generative artificial intelligence, that has begun to change.
Now, just as you can ask ChatGPT to spin up a recipe for a favorite dish or write a sonnet in the style of Lord Byron, you can now ask generative AI tools to write computer code for you. Andrej Karpathy, an OpenAI co-founder who previously led AI efforts at Tesla, recently termed this “vibe coding.”
For complete beginners or nontechnical dreamers, writing code based on vibes—feelings rather than explicitly defined information—could feel like a superpower. You don’t need to master programming languages or complex data structures. A simple natural language prompt will do the trick.
Empathy, the ability to understand what others are feeling and emotionally connect with their experiences, can be highly advantageous for humans, as it allows them to strengthen relationships and thrive in some professional settings. The development of tools for reliably measuring people’s empathy has thus been a key objective of many past psychology studies.
Most existing methods for measuring empathy rely on self-reports and questionnaires, such as the interpersonal reactivity index (IRI), the Empathy Quotient (EQ) test and the Toronto Empathy Questionnaire (TEQ). Over the past few years, however, some scientists have been trying to develop alternative techniques for measuring empathy, some of which rely on machine learning algorithms or other computational models.
Researchers at Hong Kong Polytechnic University have recently introduced a new machine learning-based video analytics framework that could be used to predict the empathy of people captured in video footage. Their framework, introduced in a preprint paper published in SSRN, could prove to be a valuable tool for conducting organizational psychology research, as well as other empathy-related studies.
Oregon State University researchers are gaining a more detailed understanding of emissions from wood-burning stoves and developing technologies that allow stoves to operate much more cleanly and safely, potentially limiting particulate matter pollution by 95%.
The work has key implications for human health as wood-burning stoves are a leading source of PM2.5 emissions in the United States. PM2.5 refers to fine particulate matter with a diameter of 2.5 micrometers or smaller that can be inhaled deeply into the lungs and even enter the bloodstream. Exposure to PM2.5 is a known cause of cardiovascular disease and is linked to the onset and worsening of respiratory illness.
Even though a relatively small number of households use wood stoves, they are the U.S.’s third-largest source of particulate matter pollution, after wildfire smoke and agricultural dust, said Nordica MacCarty of the OSU College of Engineering.