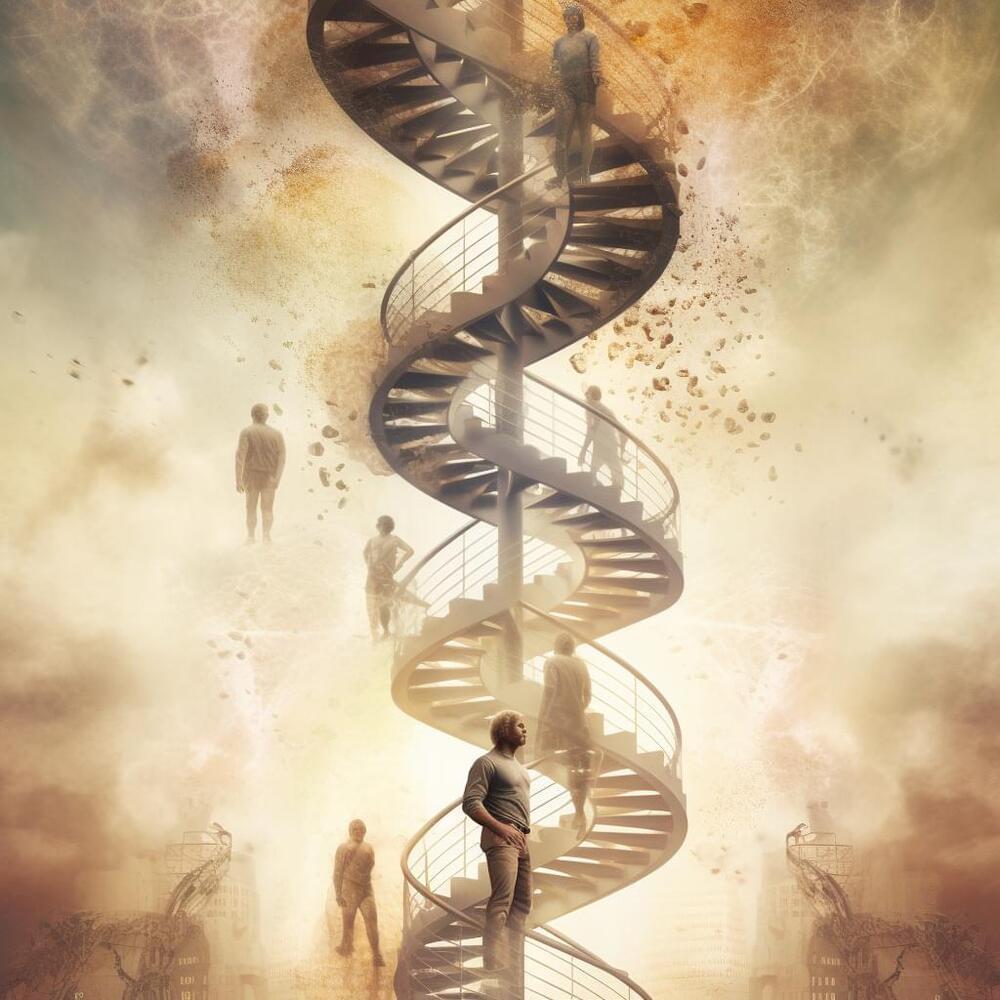
Will we ever decipher the language of molecular biology? Here, I argue that we are just a few years away from having accurate in silico models of the primary biomolecular information highway — from DNA to gene expression to proteins — that rival experimental accuracy and can be used in medicine and pharmaceutical discovery.
Since I started my PhD in 1996, the computational biology community had embraced the mantra, “biology is becoming a computational science.” Our ultimate ambition has been to predict the activity of biomolecules within cells, and cells within our bodies, with precision and reproducibility akin to engineering disciplines. We have aimed to create computational models of biological systems, enabling accurate biomolecular experimentation in silico. The recent strides made in deep learning and particularly large language models (LLMs), in conjunction with affordable and large-scale data generation, are propelling this aspiration closer to reality.
LLMs, already proven masters at modeling human language, have demonstrated extraordinary feats like passing the bar exam, writing code, crafting poetry in diverse styles, and arguably rendering the Turing test obsolete. However, their potential for modeling biomolecular systems may even surpass their proficiency in modeling human language. Human language mirrors human thought providing us with an inherent advantage, while molecular biology is intricate, messy, and counterintuitive. Biomolecular systems, despite their messy constitution, are robust and reproducible, comprising millions of components interacting in ways that have evolved over billions of years. The resulting systems are marvelously complex, beyond human comprehension. Biologists often resort to simplistic rules that work only 60% or 80% of the time, resulting in digestible but incomplete narratives. Our capacity to generate colossal biomolecular data currently outstrips our ability to understand the underlying systems.