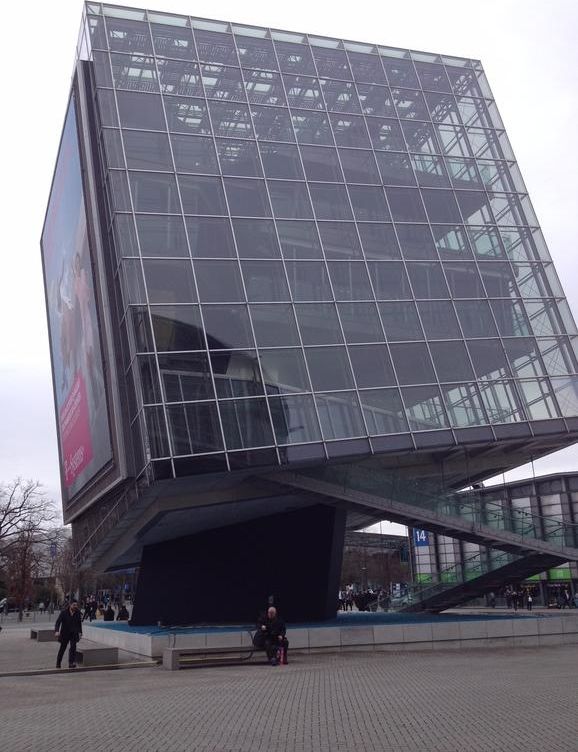
At the same time, the data that feeds AI decisions needs to be carefully vetted and reviewed. For example, “a robotic process automation solution that automates a loan process so the bank can deliver the loan faster to a client would be great,” according to Aditya Bhasin, head of consumer and wealth management technology at Bank of America, also quoted in American Banker. “But using AI or robotic process automation as a shortcut to data integration might not make sense. For example, when BofA launched a digital mortgage, ‘we could have done a whole bunch of robotics to go and pull data from different places and prepopulate the mortgage application, [but] it probably would have been fraught with error,” he said.
Too many organizations are rushing into AI without considering the full implications of the people element, according to Bessant. “It is time that we re-balance the discussion from being driven by the creators and the sellers of artificial intelligence to being balanced with the user perspective,” she says. “The discussion has been dominated by the sellers. Flip on any one of the morning financial shows and what you see is advertisement after advertisement for large and small-scale technology firms that are pushing the notion of data and modeling and that AI will help. Generally, society seems sold that artificial intelligence is better than we are as humans. However, because we build it, it is a subset of who we are and our thinking and bias.”
There’s a lot of buzz, and a lot of money now pouring into AIt’s important that some of that attention and money goes into education and building awareness of the processes behind the processes.
Read more