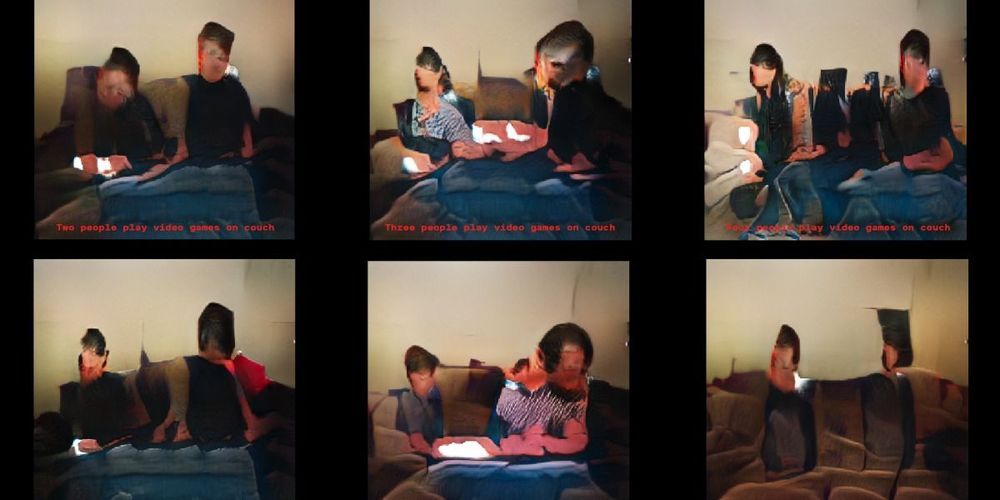
Of all the AI models in the world, OpenAI’s GPT-3 has most captured the public’s imagination. It can spew poems, short stories, and songs with little prompting, and has been demonstrated to fool people into thinking its outputs were written by a human. But its eloquence is more of a parlor trick, not to be confused with realintelligence.
Nonetheless, researchers believe that the techniques used to create GPT-3 could contain the secret to more advanced AI. GPT-3 trained on an enormous amount of text data. What if the same methods were trained on both text and images?
Now new research from the Allen Institute for Artificial Intelligence, AI2, has taken this idea to the next level. The researchers have developed a new text-and-image model, otherwise known as a visual-language model, that can generate images given a caption. The images look unsettling and freakish—nothing like the hyperrealistic deepfakes generated by GANs —but they might demonstrate a promising new direction for achieving more generalizable intelligence, and perhaps smarter robots as well.