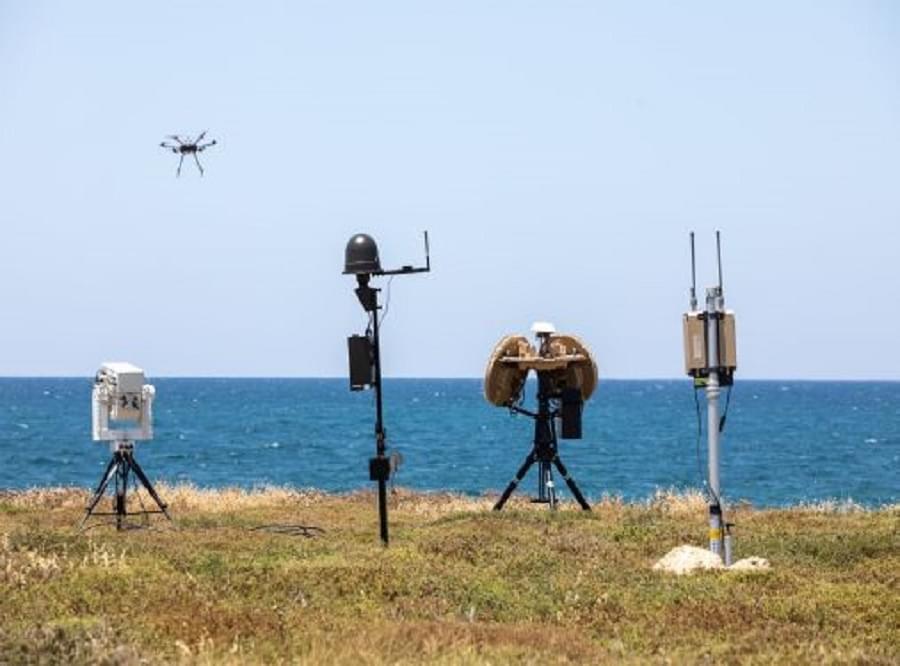
Earlier this year, in June 2,021 the British Ministry of Defence employed Rafael’s DRONE DOME counter-UAV system to protect world leaders during the G7 Summit in Cornwall, England from unmanned aerial threats. Three years ago, Britain’s Defence Ministry purchased several DRONE DOME systems which it has successfully employed in a multitude of operational scenarios, including for protecting both the physical site and participants of this year’s G7 summit. Rafael’s DRONE DOME is an innovative end-to-end, combat-proven counter-Unmanned Aerial System (C-UAS), providing all-weather, 360-degree rapid defence against hostile drones. Fully operational and globally deployed, DRONE DOME offers a modular, robust infrastructure comprised of electronic jammers and sensors and unique artificial intelligence algorithms to effectively secure threatened air space.
Meir Ben Shaya, Rafael EVP for Marketing and Business Development of Air Defence Systems: Rafael today recognizes two new and key trends in the field of counter-UAVs, both of which DRONE DOME can successfully defend against. The first trend is the number of drones employed during an attack, and the operational need to have the ability counter multiple, simultaneous attacks; this is a significant, practical challenge that any successful system must be able to overcome. The second trend is the type of tool being employed. Previously, air defense systems were developed to seek out conventional aircraft, large unmanned aerial vehicles, and missile, but today these defense systems must also tackle smaller, slower, low-flying threats which are becoming more and more autonomous.