Autonomous vehicles are barreling into the rideshare market. Lyft is asking its human drivers for their collaboration in integration.
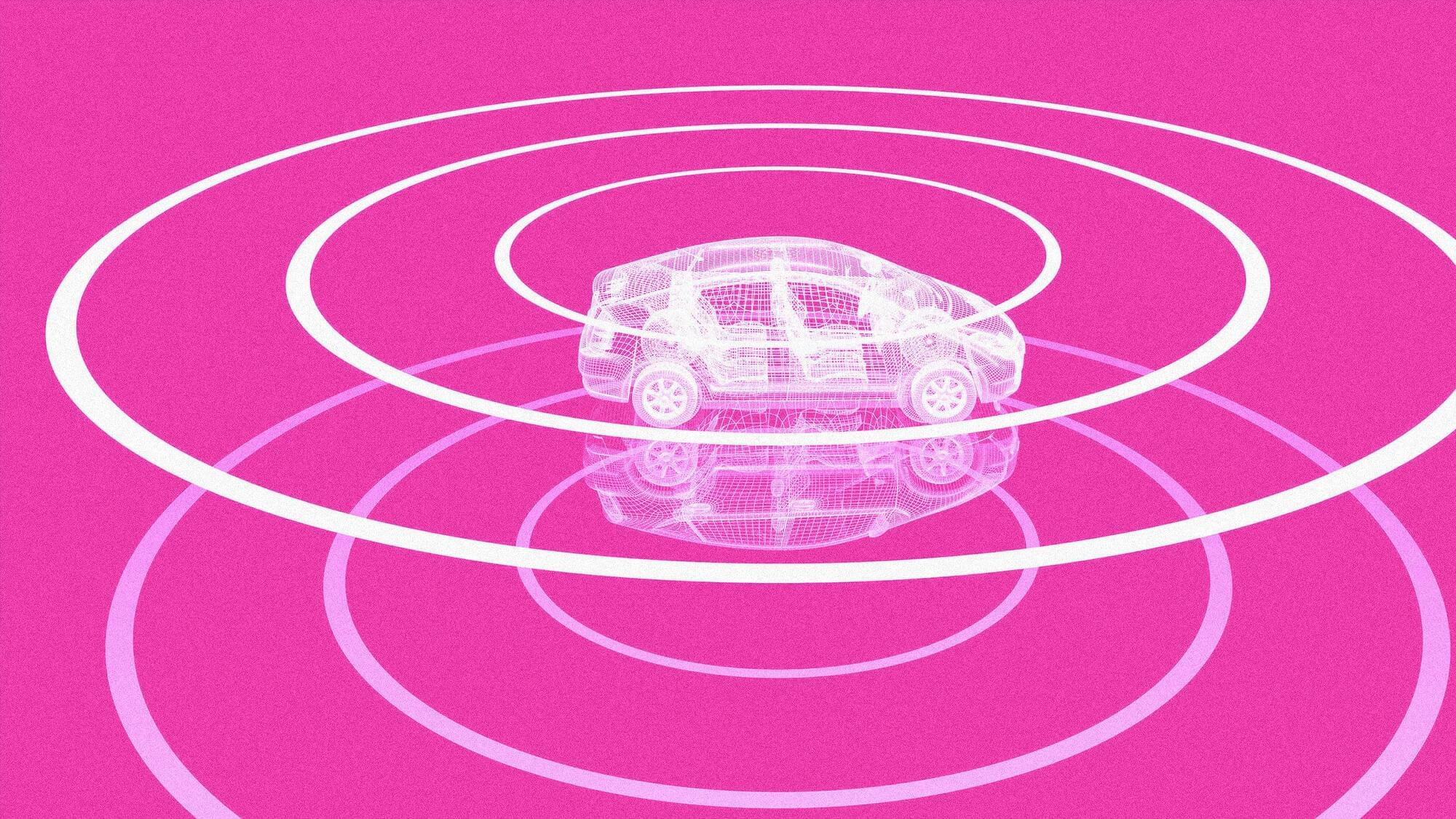
A drug commonly used to treat type 2 diabetes may reduce excess fluid in the brains of patients with hydrocephalus, which could help treat the disease less invasively than current treatments, according to a Northwestern Medicine study published in the Journal of Clinical Investigation.
Stephen Magill, MD, Ph.D., assistant professor of Neurological Surgery, was senior author of the study.
Normal pressure hydrocephalus occurs when excess cerebrospinal fluid builds up inside the skull and puts pressure on the brain. The cause of the condition is elusive and affects up to 3% of individuals over the age of 65, with symptoms including cognitive decline, difficulty walking and bladder problems.
The researchers analyzed data from the Low Frequency Array (LOFAR) radio telescope, a vast network of over 100,000 small antennas spanning eight European countries.
While studying a galaxy cluster named SpARCS1049, the researchers detected a faint, widespread radio signal. They found that it did not emanate from individual galaxies, but from a vast region of space filled with high-energy particles and magnetic fields.
Stretching over a million light-years, this diffuse glow is a telltale sign of a mini-halo, a structure astronomers have only been able to observe in the nearby universe up until now.
A new study, published in Nature Nanotechnology and featured on the journal’s front cover this month, has uncovered insights into the tiny structures that could take solar energy to the next level.
Researchers from the Department of Chemical Engineering and Biotechnology (CEB) have found that dynamic nanodomains within lead halide perovskites—materials at the forefront of solar cell innovation—hold a key to boosting their efficiency and stability. The findings reveal the nature of these microscopic structures, and how they impact the way electrons are energized by light and transported through the material, offering insights into more efficient solar cells.
The study was led by Milos Dubajic and Professor Sam Stranks from the Optoelectronic Materials and Device Spectroscopy Group at CEB, in collaboration with an international network, with key contributions from Imperial College London, UNSW Sydney, Colorado State University, ANSTO Sydney, and synchrotron facilities in Australia, the UK, and Germany.
An international team of astronomers has employed various satellites and ground-based telescopes to perform multiwavelength observations of a supernova remnant known as SNR J0450.4−7050. Results of the observational campaign, published June 18 on the pre-print server arXiv, yield new insights into the properties of this remnant, finding that it is much larger than previously thought.
Supernova remnants (SNRs) are diffuse, expanding structures resulting from a supernova explosion, which usually last several hundred thousand years before dispersing into the interstellar medium (ISM). Observations show that SNRs contain ejected material expanding from the explosion and other interstellar material that has been swept up by the passage of the shockwave from the exploded star.
Studies of SNRs beyond the Milky Way are crucial for understanding their feedback in different evolutionary phases and gaining insights into their local ISM. The Large Magellanic Cloud (LMC) is one of the galaxies that has its SNR population explored in detail.
Urea is considered a possible key molecule in the origin of life. ETH researchers have discovered a previously unknown way in which this building block can form spontaneously on aqueous surfaces without the need for any additional energy.
Urea is one of the most important industrial chemicals produced worldwide. It is used as a fertilizer, for the production of synthetic resins and explosives and as a fuel additive for cleaning car exhaust gases. Urea is also believed to be a potential key building block for the formation of biological molecules such as RNA and DNA in connection with the question of the origin of life.
Until now, the origin of urea itself on early Earth has not been conclusively clarified.
In recent years, energy engineers have been working on a wide range of technologies that could help to generate and store electrical power more sustainably. These include electrolyzers, devices that could use electricity sourced via photovoltaics, wind turbines or other energy technologies to split water (H2O) into hydrogen (H2) and oxygen (O2), via a process known as electrolysis.
The hydrogen produced by electrolyzers could in turn be used in fuel cells, devices that convert the chemical energy in hydrogen into electricity without combustion and could be used to power trucks, buses, forklifts and various other heavy vehicles, or could provide back-up power for hospitals, data centers and other facilities.
Many recently designed electrolyzers prompt the splitting of water into hydrogen using a proton exchange membrane (PEM), a membrane that selectively allows protons (H+) to pass through, while blocking gases.
When people are stressed, they make riskier decisions.
The biggest factor behind this change in behavior is a decrease in “loss aversion,” according to a recent study by University of Arkansas researchers, published in the journal Psychoneuroendocrinology. Loss aversion is the human tendency to be more affected by loss than gain. In other words, the pain of losing $100 is greater than the joy of winning $100.
The researchers also discovered that the effects of stress are different for men and women. In general, stress has a greater impact on men’s decision-making. Under stress, women are also better at predicting the outcome of a decision, while men have a better grasp of the consequences of that outcome.