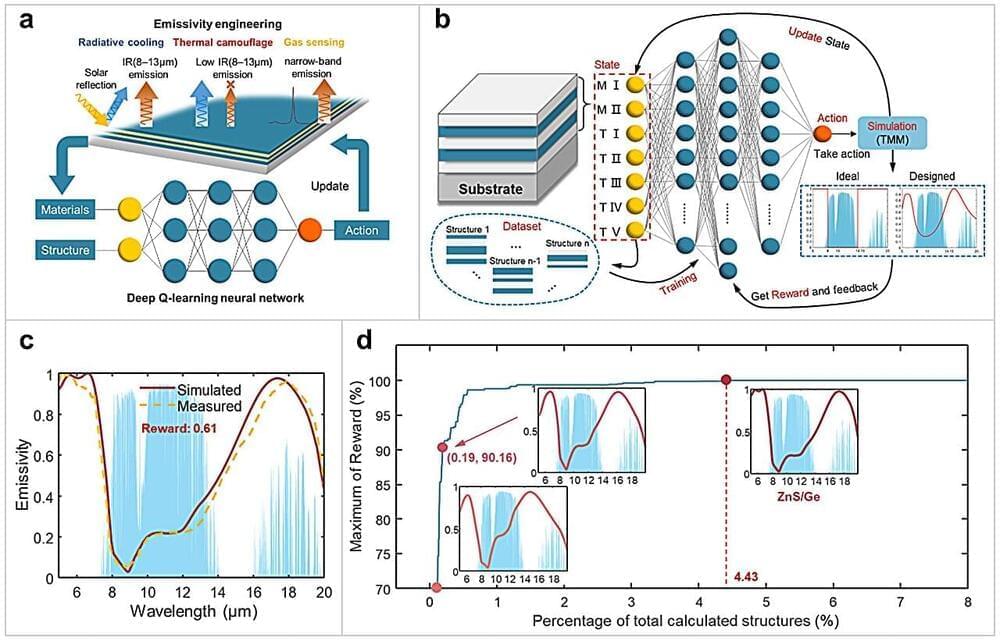
Wavelength-selective thermal emitters (WS-TEs) have been frequently designed to achieve desired target emissivity spectra, as in typical emissivity engineering, for broad applications such as thermal camouflage, radiative cooling, and gas sensing, etc.
However, previous designs required prior knowledge of materials or structures for different applications, and the designed WS-TEs usually vary from application to application in terms of materials and structures, thus there is no general design framework for emissivity engineering across different applications. Moreover, previous designs fail to tackle the simultaneous design of both materials and structures, as they either fix materials to design structures or fix structures to select suitable materials.
In a new paper published in Light: Science & Applications, a team of scientists, led by Professor Run Hu from School of Energy and Power Engineering, Huazhong University of Science and Technology, China, and coworkers have proposed a general deep learning framework based on the deep Q-learning network algorithm (DQN) for efficient optimal design of WS-TEs across different applications.